Multilayer Data and Artificial Intelligence for the Delineation of Homogeneous Management Zones in Maize Cultivation
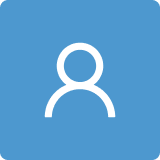
Round 1
Reviewer 1 Report
This research study the feasibility of implementing different machine learning models (supervised and unsupervised), to generate management zones in a maize plantation. The work is very interesting, well presented and developed, and in my opinion, with a few minor adjustments, it is ready to be published.
Abstract: The text should be condensed to a single paragraph to fit the journal format.
Introduction: Ensure that paragraph indentation is consistent throughout the manuscript.
Materials and Methods: Line 116-117: Add a bibliographic reference. Line 139: Clarify the meaning of BBCH. Line 193: Follow the journal's reference format guidelines.
Results: Check the numbering of the figures, as there are two figures labeled as number 5. In addition, there seems to be confusion in the text when referring to figures 5 and 6. In my understanding, figure 5 should represent León with k-means and kriging, while figure 6 should represent Zamora.bLines 275-287 could be incorporated into discussion section.
Discussion: Compare the obtained results with those of other similar investigations, such as using the kappa index, for example.
I hope these comments will help improve the quality of the work.
Author Response
I have attached a document which provides detailed responses to the comments and feedback received from reviewer 1.
Author Response File: Author Response.docx
Reviewer 2 Report
1. An overall flowchart is missed, which makes me feel difficult to understand the paper.
2. 2.2. Analysis of performance data. What is the performance data? Generally, performance indicates the accuracy and efficient of ML classification. This performance makes me confused.
3. 2.3 The data sources should be introduced before this section.
4. In my opinion, all vegetation indices are highly correlated. It is not a good choice to identify something just using VIs.
5. No significant contribution is found in this paper. In another words, the ideas, methods and findings of this paper are not novel.
6. This is not a mature manuscript for review. The English, introduction, study area, data, methods, results and discussions need to be further polished.
English is very difficult to understand/incomprehensible
Author Response
I have attached a document which provides detailed responses to the comments and feedback received from reviewer 2.
Author Response File: Author Response.docx
Reviewer 3 Report
Dear Authors
The topic is of considerable interest, as this method is faster and does not require field inspections.
The work is original as the data platforms described are of recent availability.
The experimental plan is well defined and clearly understandable
The work is clearly written and easy to read for readers with minimal knowledge of communications issues.
The conclusions reflect the initial will that the authors have set themselves.
I have some remarks:
in point 2.1 you describe the experimental sites, it would be useful, from my point of view, to integrate the description with brief references to the type of soil, the hybrid of corn used and the irrigation techniques. These data allow agronomists to better interpret the results obtained.
Author Response
I have attached a document which provides detailed responses to the comments and feedback received from reviewer 3.
Author Response File: Author Response.docx
Reviewer 4 Report
Title: “Multilayer data and artificial intelligence for the delineation of homogeneous management zones in maize cultivation” (Manuscript ID: remotesensing-2397088)
The authors of this paper aimed to estimate yield and management zones in maize plots using machine learning algorithms trained on different maize plots. While the topic is interesting, the paper has several weaknesses that need to be addressed before it can be published in the journal.
The introduction lacks essential literature and context related to the topic. It would benefit from a more detailed explanation of why the authors chose the machine learning techniques they used, as well as a discussion of other studies that employed machine learning methods to differentiate zones or areas. Furthermore, the agricultural aspects of the paper need to be strengthened.
The materials and methods section requires better information about the maize plots such as altitude, rainfall, irrigation, phenology, soil or slope; and more clarity in terms of data handling and algorithm configuration. The authors need to explain how they combined different sources of information, the types of data used, and the configuration of the algorithms. It is also unclear why the authors did not use metrics to assess the k-means clustering and how they analyzed the relationship between satellite imagery-derived maps and yield maps.
In the results section, the authors should justify the inclusion of figures 2a, 2b, and 3 (they have useful information about spatial relationships but the authors did not explain anything), and discuss their implications for model generalization. Metrics that measure the correlation between satellite-derived maps and ground truth maps are missing. The authors' statements about the patterns observed in the figures are not supported by the visuals provided. It is important to address these discrepancies and provide a more accurate interpretation of the results.
The discussion and conclusion sections need significant improvement. The authors should discuss the results and provide explanations for their findings, such as the high accuracy and Kappa coefficient values for the RF and CART models. The paper's conclusions do not align with the stated objective of generating management zones, and the authors' claim that “ML models in combination with GEE and Sentinel-2 imagery has proven to be a valuable tool for vegetative crop monitoring and the automatic generation of agricultural management area maps based on vegetation indices” is not substantiated by the results.
In conclusion, while the topic of this paper is relevant, it needs major revisions in terms of literature review, methodological clarity, result interpretation, and discussion. By addressing these issues, the authors may be able to contribute valuable insights to the field of precision agriculture and remote sensing.
Specific comments
Line 18
“This allows the creation of MZs with greater accuracy”
The results and discussion of this paper do not provide sufficient evidence to support the claim of increased accuracy in MZ creation.
Line 35
“Management zones (MZs) have …”
Management zones emerge as an outcome of implementing Site-Specific Management strategies, but there is no reference to this key concept.
Line 43
“contributing to spatial and temporal variability in the field.”
Factors contributing to spatial and temporal variability in the field have been extensively documented in the literature . Some examples (the authors may consider reading them and/or adding them to enhance their paper, but it is not mandatory):
Dhillon, Maninder Singh, Thorsten Dahms, Carina Kuebert-Flock, Thomas Rummler, Joel Arnault, Ingolf Steffan-Dewenter, and Tobias Ullmann. “Integrating Random Forest and Crop Modeling Improves the Crop Yield Prediction of Winter Wheat and Oil Seed Rape.” Frontiers in Remote Sensing 3 (January 4, 2023): 1010978. https://doi.org/10.3389/frsen.2022.1010978.
Cheng, Enhui, Bing Zhang, Dailiang Peng, Liheng Zhong, Le Yu, Yao Liu, Chenchao Xiao, et al. “Wheat Yield Estimation Using Remote Sensing Data Based on Machine Learning Approaches.” Frontiers in Plant Science 13 (December 23, 2022): 1090970. https://doi.org/10.3389/fpls.2022.1090970.
Pham, Hoa Thi, Joseph Awange, Michael Kuhn, Binh Van Nguyen, and Luyen K. Bui. “Enhancing Crop Yield Prediction Utilizing Machine Learning on Satellite-Based Vegetation Health Indices.” Sensors 22, no. 3 (January 18, 2022): 719. https://doi.org/10.3390/s22030719.
Vélez, Sergio, Florian Rançon, Enrique Barajas, Guilhem Brunel, José Antonio Rubio, and Bruno Tisseyre. “Potential of Functional Analysis Applied to Sentinel-2 Time-Series to Assess Relevant Agronomic Parameters at the within-Field Level in Viticulture.” Computers and Electronics in Agriculture 194 (March 2022): 106726. https://doi.org/10.1016/j.compag.2022.106726.
Line 44
“the lack of substantial evidence supporting the effectiveness of these techniques”
Contrary to the statement, there is a considerable body of literature supporting the effectiveness of these techniques, such as the papers listed in the previous comment. I believe that today’s challenge lies in the know-how for farmers and the costs associated with implementing these tools on the farm. The authors should conduct a more thorough literature review on this topic.
Line 48-51
“The modernization of the […] (IoT).”
The authors should expand this and provide additional references to better illustrate the role of modern technologies in agriculture. Examples of drone applications (these are examples, the authors may consider reading them and/or adding them to enhance their paper, but it is not mandatory):
Xu, Xingmei, Lu Wang, Meiyan Shu, Xuewen Liang, Abu Zar Ghafoor, Yunling Liu, Yuntao Ma, and Jinyu Zhu. “Detection and Counting of Maize Leaves Based on Two-Stage Deep Learning with UAV-Based RGB Image.” Remote Sensing 14, no. 21 (October 27, 2022): 5388. https://doi.org/10.3390/rs14215388.
Shao, Guomin, Wenting Han, Huihui Zhang, Liyuan Zhang, Yi Wang, and Yu Zhang. “Prediction of Maize Crop Coefficient from UAV Multisensor Remote Sensing Using Machine Learning Methods.” Agricultural Water Management 276 (February 2023): 108064. https://doi.org/10.1016/j.agwat.2022.108064.
Lacerda, L. N., J. Snider, Y. Cohen, V. Liakos, M. R. Levi, and G. Vellidis. “Correlation of UAV and Satellite-Derived Vegetation Indices with Cotton Physiological Parameters and Their Use as a Tool for Scheduling Variable Rate Irrigation in Cotton.” Precision Agriculture 23, no. 6 (December 2022): 2089–2114. https://doi.org/10.1007/s11119-022-09948-6.
And IoT examples (the authors may consider reading them and/or adding them to enhance their paper, but it is not mandatory):
Rezk, Nermeen Gamal, Abdel-Fattah Attia, Mohamed A. El-Rashidy, Ayman El-Sayed, and Ezz El-Din Hemdan. “An Efficient Plant Disease Recognition System Using Hybrid Convolutional Neural Networks (CNNs) and Conditional Random Fields (CRFs) for Smart IoT Applications in Agriculture.” International Journal of Computational Intelligence Systems 15, no. 1 (August 16, 2022): 65. https://doi.org/10.1007/s44196-022-00129-x.
Boursianis, Achilles D., Maria S. Papadopoulou, Panagiotis Diamantoulakis, Aglaia Liopa-Tsakalidi, Pantelis Barouchas, George Salahas, George Karagiannidis, Shaohua Wan, and Sotirios K. Goudos. “Internet of Things (IoT) and Agricultural Unmanned Aerial Vehicles (UAVs) in Smart Farming: A Comprehensive Review.” Internet of Things 18 (May 2022): 100187. https://doi.org/10.1016/j.iot.2020.100187.
Line 53
“with vegetative indices [6] being one of the most significant developments in the field.”
Vegetation indices, with over 500 in existence and dating back to the 1970s, have long been utilized in the field. The authors should explain further this statement.
Line 57
“sensor quality, leading to increased image resolution and enhanced dataset availability.”
Please, add references.
Line 58
“This progress can be attributed to various satellite sources”
Only to satellite sources? what about proximal and UAV (drone) sensing? and airborne?
Line 66-68
“SL focuses… crop monitoring”
It is unclear how the relationship between machine learning methods and the "lack of monitoring" in agricultural fields is established. Please rephrase for clarity.
Line 71
“necessary for precision agriculture”
Clarify the contribution of machine learning to precision agriculture, as the introduction appears disjointed.
line 109-113
“Then … GEE”
Provide a more detailed explanation of this section.
line 116-117
“previous studies have shown no significant improvement in results using more than three classes.”
Do you mean in maize or in agriculture in general? Please provide references to these studies.
Lines 118-120
“ranging from…12.00-30.00 t/ha”
Explain the rationale behind the chosen values.
line 130
“The GEE platform's Application Program Interface”
Specify whether Python or JavaScript was used, and elaborate further on the implementation.
Line 132
“cloud mask”
Clarify if the cloud mask was generated or if it is part of the products offered by the Copernicus system (MSK_CLASSI).
Line 134
“bitmask band with quality cloud mask information with a spatial resolution of 60 meters (QA60)”
Explain the origin of this mask and whether it was downloaded.
Line 149
“A reduction function”
Provide the equation for this function and describe it in detail.
line 153
“figure “
Elaborate further on this figure, as it is not a "methodology."
line 155
“Table 2”
The references to the vegetation indices are wrong; for example, NDVI is Rouse.
Line 162
“This function allows us to define the resolution of 10 meters.”
Explain how this is achieved.
Line 167-170
Avoid redundancy, this was previously explained.
line 169
“and the values of vegetation indices calculated from Sentinel-2 imagery”
Explain the methodology for combining different sources of information. Was it the same as in Figure 1?
Line 171
“data”
Clarify what kind of data: images, pixels, data in rows and columns (datasheet)...
Line 172-186
Provide essential information on the configuration of the algorithms, such as the number of trees in RF or the number of groups in k-means.
Line 191
“indicators, namely the overall accuracy and the Kappa coefficient, to evaluate the supervised classification”
Include the equations for these indicators.
Line 199
“a visual interpretation of the results was performed,”
Explain why metrics were not used instead of visual interpretation.
Line 218
“the RMSE values being relative to 0 and 1”
Clarify the meaning of this sentence.
line 227
Consider whether Figure 2a, 2b, and 3 are necessary.
line 231
“revealed three distinct zones”
Rephrase to acknowledge the continuous color scale present in Figure 4, rather than implying discrete zones.
line 243
“Figure 4”
Address the discrepancy between the presence of more colors and the three classes mentioned.
line 260
“the highest accuracy”
The highest accuracy was achieved in training and validation within the same plot, correct? It is consfusing.
Line 261-266
“The RF model … Variables”
Please specify the number of trees and the configured parameters for the algorithms. Additionally, consider moving this information to the Materials and Methods section.
Line 271
“all indices exhibited similar patterns”
Not all indices exhibit similar patterns; MCARI, NDVI, and SIPI show distinct differences from the rest.
line 272
“values around the plot's perimeter,”
Explain the reason for these values occurring around the plot's perimeter.
Line 273
“SIPI index identified a distinct high”
Clarify the cause of the distinct high identified by the SIPI index.
line 275-279
“These results …crop yields.”
The authors should acknowledge that the results were not successful in this instance.
Line 286
“valuable insights into the spatial variability of crop yield,”
The maps do not provide valuable insights, as they do not display consistent visual patterns, and the authors were unable to delineate different management areas effectively (according to the ground-truth data).
line 287
“identify factors contributing to yield variation,”
Specify the factors contributing to yield variation.
Line 299
“exhibited the same pattern in the perimeter zones”
Clarify that the pattern is not the same, as a "canvas" of low values is observed in some maps.
line 300
“there were differences in the interior part,”
Acknowledge that there are no discernible differences in the inside of most maps (Figure 6).
line 341-344
This information is already well-known, therefore is not a novelty of the paper.
line 353
“that are difficult to access.”
Clarify which agricultural plots (which are typically subjected to daily or weekly operations) are challenging to access under standard conditions.
Line 365-366
“GEE offers the possibility to generate zone maps automatically without the need to download the images or bands.”
Reiterate that this is a well-known aspect of GEE. It is not clear where is the novelty of the paper.
line 370
“valuable and up-to-date crop information,”
Acknowledge that the results do not support this statement.
line 377-379
“ML models in combination with GEE and Sentinel-2 imagery has proven to be a valuable tool for vegetative crop monitoring and the automatic generation of agricultural management area maps based on vegetation indices.”
Revise this statement, as the results do not support the claim that accurate maps were generated from satellite information or that management zones were delineated properly.
The paper requires a thorough revision for language and clarity, as there are numerous grammatical errors and awkward phrasings throughout the text. Some examples: line 114 "After data processing the data" and line 256 “claclassifyingng”
Author Response
I have attached a document which provides detailed responses to the comments and feedback received from reviewer 4.
Author Response File: Author Response.docx
Round 2
Reviewer 2 Report
I do not find the flowchart in my review version.
Eq.(1) and Fig.(1) are weird.
English needs to be further edit.
Reviewer 4 Report
The recent corrections to the manuscript have enhanced its readability and academic depth, effectively addressing the previously raised concerns.
Two areas require further attention prior publication. Firstly, the clarity and quality of the figures, along with their corresponding captions, need improvement:
- Captions should be self-contained, providing sufficient explanation for readers to understand the figure independently of the main text.
- Reference formatting needs to be meticulously scrutinized. Many references currently terminate with a semicolon, and contain incomplete bibliographic information.
In addition, a careful revision of the text is warranted to avoid errors and confusions such as “vegetative indices” instead of “vegetation indices”.
Several instances of English language errors have been noted, which could affect the readability and overall professional tone of the manuscript. These errors should be rectified prior to resubmission (some examples: "Bigdata" should be "big data", "patrons" should be "patterns").