Chemometric Assessment of Soil Pollution and Pollution Source Apportionment for an Industrially Impacted Region around a Non-Ferrous Metal Smelter in Bulgaria
Abstract
: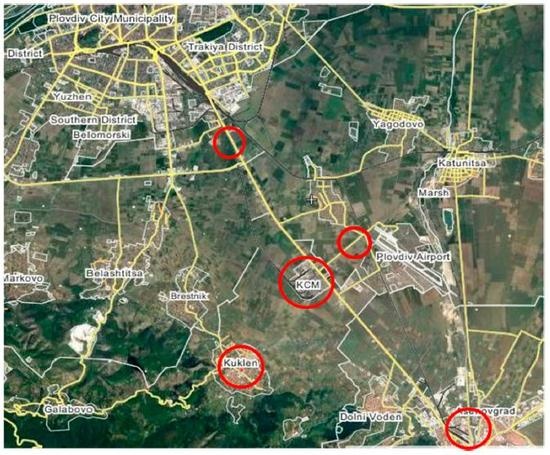
1. Introduction
2. Input Data Set
2.1. Objects
2.2. Cheimical Variables
3. Results and Discussion
3.1. Cluster Analysis
3.1.1. Clustering of the Variables
3.1.2. Clustering of the Objects
- C1 (KRM_10, KRM_11, KRM_12, PB_10, PB_11, PB_12)
- C2 (KAGRI_10, KAGRI_11, KAGRI_12, AS_10)
- C3 (K_10, K_11, K_12, PBUR)
- C4 (AS_11, AS_12)
3.2. Principal Components Analysis
3.3. Source Apportionment
3.4. Comparison of the Metal Levels of Pollution in the Soil in the Vicinity of the Nonferrous Smelter for the Period 2000–2012
4. Materials and Methods
4.1. Sample Collection, Preparation and Chemical Analysis
4.2. Chemometric Methods
4.2.1. Cluster Analysis
4.2.2. Principal Components Analysis
4.2.3. Source Apportionment
5. Conclusions
- By means of the source apportionment model and the average of the concentrations measured we have proven another source of copper impact different from the smelter itself; the copper concentration at the smelter sampling point is the lowest;
- Despite the fact that the smelter continues to be the major source of Zn, Cd, Hg and to lesser extent Pb pollution, comparison to previous data with these ones shows a significant decrease of the emissions of Zn, Cd and Pb;
- Though our results, do not relate the smelter production to copper pollution, it is readily seen that the copper content in the soil is drastically reduced. One of the reasons is the closure of the mine in the village of Zvezdel and the exchange of the ore source with another one. Another possible reason is that the copper source treated by us as geogenic and is related to the ore production and flotation around the mines near the settlements of Luki, Madan and Madjarovo;
- The higher levels of pollution at location AS (town of Assenovgrad) are due to the natural contribution of the underground waters and the Chaia River carrying waste from the lead-zink rich ores of Rhodopa Mountain where the mines and flotation facilities are located. The multivariate statistical expertise indicates this specificity by the contribution of the “natural source” in the apportionment models.
Supplementary Materials
Author Contributions
Funding
Acknowledgments
Conflicts of Interest
References
- Bacon, J.R.; Dinev, N.; Stanislavova, L.; Penkov, D.; Willeke-Wetstein, C. The route of transfer to the human population of lead from contaminated soil close to a smelter in Bulgaria. J. Phys. IV France 2003, 107, 91–94. [Google Scholar] [CrossRef]
- Bacon, J.R.; Dinev, N.S. Isotopic characterisation of lead in contaminated soils from the vicinity of a non-ferrous metal smelter near Plovdiv, Bulgaria. Environ. Pollut. 2005, 134, 247–255. [Google Scholar] [CrossRef] [PubMed]
- Zhang, X.; Yang, L.; Li, Y.; Li, H.; Wang, W.; Ge, Q. Estimation of lead, and zinc emissions from mineral expoitation based on characteristics of lead/zinc deposits in China. Trans. Nonferrous Met. Soc. China 2011, 211, 2513–2519. [Google Scholar] [CrossRef]
- Vrhovnik, P.; Dolenec, M.; Serafimovski, T.; Tasev, G.; Arrebola, J.P. Assessment of essential and nonessential dietary exposure to trace elements from homegrown foodstuffs in a polluted area in Makedonska Kamenica and the Kočani region (FYRM). Sci. Total Environ. 2016, 559, 204–211. [Google Scholar] [CrossRef] [PubMed] [Green Version]
- Woszczyk, M.; Spychalski, W.; Boluspaeva, L. Trace metal (Cd, Cu, Pb, Zn) fractionation in urban-industrial soils of Ust-Kamenogorsk (Oskemen), Kazakhstan—implications for the assessment of environmental quality. Environ. Monit. Assess. 2018, 190, 362–377. [Google Scholar] [CrossRef] [PubMed]
- Zhan, H.Y.; Jiang, Y.F.; Yuan, J.W.; Hu, X.F.; Nartey, O.D.; Wang, B.L. Trace metal pollution in soil and wild plants from lead-zinc smelting areas in Huixian County, northwest China. J. Geochem. Explor. 2014, 147, 182–188. [Google Scholar] [CrossRef]
- Zheng, N.; Wang, Q.; Zheng, D. Health risk of Hg, Pb, Cd, Zn, and Cu to the inhabitants around Huludao Zinc Plant in China via consumption of vegetables. Sci. Total Environ. 2007, 383, 81–89. [Google Scholar] [CrossRef] [PubMed]
- Zhuang, P.; McBride, M.B.; Xia, H.; Li, N.; Li, Z. Health risk from heavy metals via consumption of food crops in the vicinity of Dabaoshan mine, South China. Sci. Total Environ. 2009, 407, 1551–1561. [Google Scholar] [CrossRef] [PubMed]
- Xu, D.; Zhou, P.; Zhan, J.; Gao, Y.; Dou, C.; Sun, Q. Assessment of trace metal bioavailability in garden soils and health risk svia consumption of vegetables in the vicinity of Tongling mining area, China. Ecotox. Environ. Safe. 2013, 90, 103–111. [Google Scholar] [CrossRef] [PubMed]
- Zeng, F.; Ali, S.; Zhang, H.; Ouyang, Y.; Qiu, B.; Wu, F.; Zhang, G. The influence of pH and organic matter content in paddy soil on heavy metal availability and their uptake by rice plants. Environ. Pollut. 2011, 159, 84–91. [Google Scholar] [CrossRef] [PubMed]
- Bi, C.; Zhou, Y.; Chen, Z.; Jia, J.; Bao, X. Heavy metals and lead isotopes in soils, road dust and leafy vegetables and health risks via vegetable consumption in the industrial areas of Shanghai, China. Sci. Total Environ. 2018, 619–620, 1349–1357. [Google Scholar] [CrossRef] [PubMed]
- Ettler, V. Soil contamination near non-ferrous metal smelters: A review. Appl. Geochem. 2016, 64, 56–74. [Google Scholar] [CrossRef] [Green Version]
- Kang, M.J.; Kwon, Y.K.; Yu, S.; Lee, P.K.; Park, H.S.; Song, N. Assessment of Zn pollution sources and apportionment in agricultural soils impacted by a Zn smelter in South Korea. J. Hazard. Mater. 2019, 364, 475–487. [Google Scholar] [CrossRef] [PubMed]
- Sun, J.; Yu, R.; Hu, G.; Su, G.; Zhang, Y. Tracing of heavy metal sources and mobility in a soil depth profile via isotopic variation of Pb and Sr. CATENA 2018, 171, 440–449. [Google Scholar] [CrossRef]
- Jeon, S.K.; Kwon, M.J.; Yang, J.S.; Lee, S. Identifying the source of Zn in soils around a Zn smelter using Pb isotope ratios and mineralogical analysis. Sci. Total Environ. 2017, 601–602, 66–72. [Google Scholar] [CrossRef] [PubMed]
- Beata, L.; Cezary, K.; Jaroslaw, W. Ambient geochemical baselines for trace elements in Chernozems-approximation of geochemical soil transformation in an agricultural area. Environ Monit Assess. 2018, 191, 19. [Google Scholar] [CrossRef] [PubMed]
- Yang, Y.; Christakos, G.; Guo, M.; Xiao, L.; Huang, W. Space-time quantitative source apportionment of soil heavy metal concentration increments. Environ. Pollut. 2017, 223, 560–566. [Google Scholar] [CrossRef] [PubMed]
- Ma, W.; Tai, L.; Qiao, Z.; Zhong, L.; Wang, Z.; Fu, K.; Chen, G. Contamination source apportionment and health risk assessment of heavy metals in soil around municipal solid waste incinerator: A case study in North China. Sci. Total Environ. 2018, 631–632, 348–357. [Google Scholar] [CrossRef] [PubMed]
- Bojinova, P.; Georgiev, B.; Kabakchiev, I.; Krasteva, V.; Stanislavova, L.; Tchuldjian, H.; Welp, G.; Brümmer, G.W. Harmonization of the Methods for the Investigation of Heavy Metal Pollution of Soils and the Standardization of the Assessment Criteria for Soil Protection; Report No. UBA-FB 96–071; Umweltbundesamt: Berlin, Germany, 1996. [Google Scholar]
- Sengalevich, G. Problems of pollution with heavy metals and land usage of agricultural lands in the region of KZM-Plovdiv. Ecobulletin 1999, 1, 10–17. (in Bulg). [Google Scholar]
- De Temmerman, L.; Waegeneers, N.; Claeys, N.; Roekens, E. Comparison of concentrations of mercury in ambient air to its accumulation by leafy vegetables: An important step in terrestrial food chain analysis. Environ. Pollut. 2009, 157, 1337–1341. [Google Scholar] [CrossRef] [PubMed]
- Reid, R.; Hayes, J.; Kwang, W.J. Mechanisms and Control of Nutrient Uptake in Plants. Int. Rev. Cytol. 2003, 229, 73–114. [Google Scholar] [CrossRef] [PubMed]
- Pacyna, E.G.; Pacyna, J.M.; Pirrone, N. European emissions of atmospheric mercury from anthropogenic sources in 1995. Atmos. Environ. 2001, 35, 2987–2996. [Google Scholar] [CrossRef]
- Thurston, G.D.; Spengler, J.D. A quantitative assessment of source contributions to inhalable particulate matter pollution in metropolitan Boston. Atmos. Environ. 1985, 19, 9–25. [Google Scholar] [CrossRef]
- Vitov, O.; Marinova, I. Distribution of cinnabar (HgS) in alluvial sediments in Bulgaria. Compt. Rend. Acad. Bul. Sci. (Geologie) 2005, 58, 1287–1290. [Google Scholar]
- Soil Quality—Determination of pH; ISO 10390:2005; ISO Technical Committee ISO/TC 190-SC 3: Bad Mergentheim, Germany, 2005.
- Soil Quality—Determination of Carbonate Content—Volumetric Method; ISO 10693:1995; ISO Technical Committee ISO/TC 190-SC 3: Bad Mergentheim, Germany, 1995.
- Soil Quality—Determination of Organic and Total Carbon after Dry Combustion (Elementary Analysis); ISO 10694:1995; SO Technical Committee ISO/TC 190-SC 3: Bad Mergentheim, Germany, 1995.
- International Organization for Standardization. Soil Quality—Extraction of Trace Elements Soluble in Aqua Regia; ISO 11466:1995; ISO Technical Committee ISO/TC 190-SC 3: Bad Mergentheim, Germany, 1995. [Google Scholar]
- Hoffman, G.L. Methods of Analysis by the U.S. Geological Survey National Water Quality Laboratory—Preparation Procedure for Aquatic Biological Material Determined for Trace Metals; Open-File Report 96-362; U.S. Geological Survey, Branch of Information Services: Denver, CO, USA, 1996. [CrossRef]
- Massart, D.L.; Kaufman, L. The Interpretation of Analytical Chemical Data by the Use of Cluster Analysis; John Wiley & Sons: New York, NY, USA, 1983. [Google Scholar]
Sample Availability: Not available. |
Groups of Variables | Variables | Notation, e.g., |
---|---|---|
soil structural (Hh, CEC, TEB) and physicochemical parameters | hydraulic activity; soil capacity; base exchange capacity; organic carbon; total organic carbon; pH, measured in KCl extract; pH, measured in water extract; saturation | Hh CEC TEB C_org TOC pH_KCl pH_w V |
soil nutritional components | Mg, P, K, N | Mg_bio; N |
soil pollution by heavy metals | Hg, Cd, Zn, Cu, Pb | Hg_soil |
cabbage leaves pollution by heavy metals | Hg, Cd, Zn, Cu, Pb | Hg_leavs |
Factor Loadings (Varimax normalized) (Marked loadings are >statistically significant) | |||||
Variable | PC1 | PC2 | PC3 | PC4 | PC5 |
pH_KCl | 0.217 | 0.009 | 0.919 | 0.182 | 0.169 |
pH_w | 0.171 | 0.118 | 0.915 | 0.122 | 0.257 |
C_org | 0.974 | 0.016 | 0.119 | −0.036 | 0.020 |
TOC | 0.974 | 0.016 | 0.119 | −0.036 | 0.020 |
N | 0.943 | −0.106 | 0.159 | 0.069 | −0.065 |
P_bio | −0.055 | 0.275 | 0.107 | 0.852 | 0.036 |
K_bio | −0.308 | −0.046 | 0.274 | −0.081 | 0.610 |
Mg_bio | 0.078 | −0.291 | 0.480 | 0.807 | −0.005 |
Zn_soil | −0.030 | 0.876 | −0.040 | 0.118 | 0.357 |
Cd_soil | −0.190 | 0.903 | 0.043 | −0.150 | −0.282 |
Pb_soil | −0.069 | 0.442 | −0.496 | 0.593 | 0.258 |
Cu_soil | 0.173 | −0.022 | 0.128 | 0.127 | 0.893 |
Hg_soil | 0.202 | 0.812 | 0.085 | 0.416 | −0.177 |
Expl. Var % | 23.7 | 20.2 | 17.9 | 15.5 | 12.1 |
Variable | Unidentified Sources (Intercept) | Organic Source | Non-Ferrous Smelter Source | Acidic Source | Second Anthropogenic Contribution Source | Natural Source | R2 |
---|---|---|---|---|---|---|---|
pH_KCl | 4.7 | 8.6 | - | 78.6 | 4.3 | 3.8 | 0.84 |
pH_w | 3.2 | 9.1 | - | 80.2 | 4.1 | 3.4 | 0.84 |
C_org | 3.4 | 96.6 | - | - | - | - | 0.87 |
TOC | 3.2 | 96.8 | - | - | - | - | 0.88 |
N | 11.4 | 88.6 | - | - | - | - | 0.92 |
P_bio | 8.6 | - | 15.9 | - | 75.5 | - | 0.82 |
K_bio | 10.4 | 12.8 | - | 9.1 | - | 67.7 | 0.81 |
Mg_bio | 7.3 | - | - | 10.6 | 82.1 | - | 0.82 |
Zn_soil | 0.9 | - | 91.6 | - | - | 7.5 | 0.92 |
Cd_soil | 5.4 | - | 82.9 | - | 5.6 | 6.1 | 0.88 |
Pb_soil | 3.8 | - | 21.1 | 3.2 | 61.7 | 10.2 | 0.89 |
Cu_soil | 9.3 | - | - | 3.3 | 3.1 | 84.3 | 0.93 |
Hg_soil | 5.0 | - | 90.6 | - | 4.4 | - | 0.84 |
Locations | Metal Concentrations in the Topsoils (0–20) [mg kg−1] | Ref. | ||||
---|---|---|---|---|---|---|
Zn | Cd | Pb | Cu | Hg | ||
K * | 103.74 | 0.17 | 17.66 | 33.55 | 0.033 | present |
Kuklen | 158 | 3 | 97 | 49 | - | [2] |
KRM * | 66.33 | 0.38 | 14.33 | 12.07 | 0.036 | present |
KAGRI * | 384.60 | 2.22 | 19.69 | 8.00 | 0.106 | present |
Kuklen around smelter | 5917 | 138 | 4892 | 666 | - | [2] |
AS * | 409.70 | 0.97 | 31.68 | 25.22 | 0.072 | present |
Boyntsi (14 km SE of Kuklen) | 340 | 5 | 225 | 71 | - | [2] |
PB * | 330.40 | 0.81 | 14.73 | 30.76 | 0.043 | present |
© 2019 by the authors. Licensee MDPI, Basel, Switzerland. This article is an open access article distributed under the terms and conditions of the Creative Commons Attribution (CC BY) license (http://creativecommons.org/licenses/by/4.0/).
Share and Cite
Dimitrov, D.S.; Nedyalkova, M.A.; Donkova, B.V.; Simeonov, V.D. Chemometric Assessment of Soil Pollution and Pollution Source Apportionment for an Industrially Impacted Region around a Non-Ferrous Metal Smelter in Bulgaria. Molecules 2019, 24, 883. https://doi.org/10.3390/molecules24050883
Dimitrov DS, Nedyalkova MA, Donkova BV, Simeonov VD. Chemometric Assessment of Soil Pollution and Pollution Source Apportionment for an Industrially Impacted Region around a Non-Ferrous Metal Smelter in Bulgaria. Molecules. 2019; 24(5):883. https://doi.org/10.3390/molecules24050883
Chicago/Turabian StyleDimitrov, Dimitar S., Miroslava A. Nedyalkova, Borjana V. Donkova, and Vasil D. Simeonov. 2019. "Chemometric Assessment of Soil Pollution and Pollution Source Apportionment for an Industrially Impacted Region around a Non-Ferrous Metal Smelter in Bulgaria" Molecules 24, no. 5: 883. https://doi.org/10.3390/molecules24050883
APA StyleDimitrov, D. S., Nedyalkova, M. A., Donkova, B. V., & Simeonov, V. D. (2019). Chemometric Assessment of Soil Pollution and Pollution Source Apportionment for an Industrially Impacted Region around a Non-Ferrous Metal Smelter in Bulgaria. Molecules, 24(5), 883. https://doi.org/10.3390/molecules24050883