Earthquake Nowcasting with Deep Learning
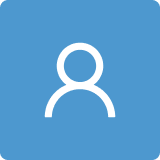
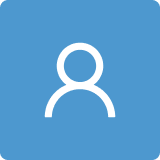
Round 1
Reviewer 1 Report
The paper, although tackling the problem in a novel an innovate manner, needs some major revisions with regards to presentation and clarity. Formatting of equations and figures make some sections uninterpretable. I would recommend typsetting equations using LaTeX and ensuring that the text in figures is large enough so that it can be read. The section 'The Deep Learning Inputs and Outputs' is crucial for the reader to understand how the proposed models are implemented, however its writing style is cluttered and could be summarised with a more concise paragraph or appropriate equation. This approach could be applied to many of the other sections.
The methodology is generally sound, although I had some concerns with the partitioning of the testing and training datasets. Particularly, since the partitioning is done randomly in space, one would expect significant correlation between training pixels and testing/validation pixels, and so the reported Normalized Nash Sutcliffe Efficiency for validation is not on 'completely' unseen data. Furthermore, the results presented only compare across their proposed models and do not present how well a baseline control model would perform.
Author Response
Please see the attachment.
Author Response File: Author Response.pdf
Reviewer 2 Report
The paper presents an exercise to implement deep learning techniques to earthquake datasets to nowcast earthquakes. The paper uses the implementation of some new fancy and computationally intensive techniques to improve the previous results of their own work. Personally, I am also very much fond of using new machine learning techniques to explore new meanings for earthquake forecasting. However, this field of work should not be treated as just playing with data to get some numbers (performance metrics).
When we subject some data to machine learning, it will yield some outputs and some outputs will be better than others. However, the foremost thing is that problem must be formulated in a proper way so that it can represent a real problem. Only then the performance metrics can mean something or may lead to useful research if the problem formulation is driven by the needs of society or real issue.
To my understanding, the authors divide California into 0.1x0.1 grid and use data of some cells to train the model and then try to forecast/nowcast earthquakes for some other cells. In my opinion, this is not a real problem. As a student of earthquake forecast research, I feel that this is not how the real problem is. We are not interested in forecasting/nowcasting earthquakes in a single cell or 11x11km for the whole duration of time and leaving all the other cells out of it. The performance results and fancy techniques come later after the problem is formulated correctly.
In my opinion, the problem should be formulated in such a way to forecast along time. Use data from the past to model and predict the future. So the idea problem formulation includes discretizing spatial region into 0.1x0.1 cells and then using all the data of the cells from the past to forecast earthquakes future earthquakes for each cell in the future. Then the paper will be addressing a real significant scientific problem.
Some minor suggestions:
- The font size of labels should be increased.
- The captions should be self-explanatory and covey meaningful information.
- Define radius of Gyration in text. I could not find the definition of this term.
- The term "Multiplicity" refers to number of earthquakes, as per my understanding. If I am right, then why not use a simple term like N_eqs or just N?
- In Table 1, Full Multiplicity is not explicitly defined. Although, I understood the meaning but should be clearly mentioned.
- On page 10, it talks about faults. My question is, who marked these faults? Someone must have done it before or do authors define them by themselves? The suitable literature must be referred here that marks these faults.
- Lastly, why authors thought about using a 0.1x0.1 degree grid. I assume that the most probable reason is that it is straightforward to implement and easy to understand. But it is better if authors highlight the reason in their words.
I hope the authors find my comments useful.
Best wishes
Author Response
Please see the attachment.
Author Response File: Author Response.pdf
Reviewer 3 Report
This is a paper focusing on Earthquake Nowcasting with the use of Deep Learning techniques. In principle the paper is interesting and there is no doubt that a lot of work has been done. However, the paper suffers from several points of view, which can be summarized as follows:
- Organization and presentation of the paper,
- Literature and citations,
- Many technical issues.
Due to these serious disadvantages the paper cannot be accepted as it is. There is need for drastic improvement and reconsideration after major revision.
- Organization and presentation of the paper.
- In many points of the paper it is not clear what are the innovative aspects introduced and which are the approaches already used in previous papers. This needs clarification.
(1) Section “1. Introduction” is too long. I recommend to shorten it by focusing only in explaining briefly the scope of the paper and how it is facilitated by the data, methods and techniques used. Then, the sub-section 1.1 “Introduction to Earthquake Nowcasting’ should be placed as a 2nd Section titled something like “2. Earthquake Nowcasting: A short overview”. Also, the sub-section 1.2 “Research objectives” (l. 168-182) is referring to techniques used, therefore it is out of place in the Introduction.
(2) The methodology section is missing from the paper but it is needed. The authors should create a new Section “3. Methods and Data”, which could comprise “3.1 Data” from currently l.183 to l.193, and “3.2 Methods and Techniques” that could include the part “Research objectives” (currently l. 168-182) as well as all the sub-sections from currently l. 194 to l. 507 rearranged in appropriately numbered sub-sections.
(3) Section “5. Initial Earthquake Nowcasting results” should be numbered as 4, while “6. Conclusions” should be “5. Discussion and Conclusions”.
(4) The paper presentation suffers from the fact in the text Figures as a rule precede the relevant paragraphs. The authors should put figures after the relevant paragraphs.
(5) Tables 2,3 and 4 are not referred to into the text.
(6) In figures Fig 5a,b,c,d, 6, 7,11,12, 16-19 the fonts are too small making them unreadable.
(7) There is need for a map plotting the study area, the large earthquakes which are illustrated in the other figures as well as the known faults in the study area.
- Literature and citations need significant improvement. For example, please avoid using Wikipedia as much as possible. It is preferable to rather use initial scientific papers, e.g. for the Nash Sutcliffe Efficiency as reference [34] use the paper by Nash, J. E. & Sutcliffe, J. V. (1970). "River flow forecasting through conceptual models part I — A discussion of principles". Journal of Hydrology. 10 (3): 282–290, where this efficiency metric was introduced.
Also, for “Benioff Strain” (l. 224) do not quote other papers using this metric (i.e. [39]–[42]) but quote the original paper(s) by Benioff who introduced the concept.
- Technical issues
31. Replace risk by Hazard.
107. Km instead of KM.
111. Radius of Gyration of Bursts. Please complete or omit the sentence.
187. “…present day…”. Please clarify what present day means.
187. “…ground location…”. Do you mean “epicenter”?
192. “…known faults…”. The authors should explain the source of the data set they used for “…known faults…”.
195. “…we calculate summed magnitudes…”. Please explain what “summed magnitudes” means.
197. “…using Energy ~ 101.5m…” . Please explain which formula has been used, provide citation.
204. Please better explain quantity Q.
218. “ …deep learning values of O(1)…”. Please explain O(1).
234.Table 1. It is cut at the right hand side.
275. “a static label from 0..35”. Perhaps 0.35, correct?
358. “In the Covid example illustrated in fig. 3…”. This is a confusing statement. Fig. 3 does not show something about Covid. The only relevant is Figure 12, but it appears after l. 413.
424. Legend of Fig. 13. The statement “The latter has an attention-based decoder and an LSTM-….” looks like incomplete.
441. “…for the encoder as shown in fig. 5b..”. Fig. 5b does not show that, please check.
442. “We compare the two architectures shown in a) and b) of fig. 13; the 442 pure LSTM used in sec. 2 and the science transformer”. This sentence looks like incorrect, please check.
473-474. “The next subsection describes the 3 search strategies we have 473 looked at: and depicted in fig. 15”. Please correct as follows: “The next subsection describes the three search strategies we have looked at and depicted in fig. 15”.
Author Response
Please see the attachment.
Author Response File: Author Response.pdf
Reviewer 4 Report
Dear Editor,
I have reviewed the manuscript “ Earthquake Nowcasting with Deep Learning”, by G. Fox, J. Rundle, A. Donnellan and B. Feng.
This work presents deep learning approaches for estimating the seismic risk in the immediate past, present and near future, that is the concept of nowcasting.
The paper’s goal is well delineated and very interesting.
The paper is well written in the initial part, but then, since more approaches are considered, it is not easy to follow all the sections.
- The prediction concerns time and space information. However, it is not clear to me, what is actually predicted and with which time in advance. Is it the maximum magnitude in a given place at a given time with a time period in advance predicted?
- When a 208 weeks period is considered, is it both for the past and the future?
- My main concern is about the section describing results. After 19 pages of description of data, methods and so on, at the end the results are summarized in just less than a single page of text. I think this is pity, because as reader I would expect a deeper description of results, a discussion of advantages and drawbacks of different approaches. The description of results is so synthetic that, honestly, I cannot fully understand them. I see from table 5 that scores are vey high, but what is predicted?
I am confident that with a little work on the text to make the paper more understandable, the work can be ready for publication. I suggest moderate revision.
Sincerely
Author Response
Please see the attachment.
Author Response File: Author Response.pdf
Round 2
Reviewer 1 Report
It is my belief that the construction of the experiment does not demonstrate the effectiveness of the introduced models in a manner that is scientifically sound. Although it is mentioned in the 'Conclusions' section that the Authors would like to investigate whether their choice of testing/training set affects their results in the future, testing their models on data that is highly correlated with their training data invalidates the conclusion that this paper draws. My suggestion would be to reconstruct the experiment to remove this spatial correlation. This can be done either by a sensible partitioning in time or reporting scores from a 'Spatial Cross Validation'.
Meyer, Hanna, et al. "Importance of spatial predictor variable selection in machine learning applications–Moving from data reproduction to spatial prediction." Ecological Modelling 411 (2019): 108815.
Furthermore, I would suggest making sure all figures are clear enough so that text is not pixelated.
Author Response
We agree that final conclusions need a more thorough examination of the validation and testing process. However, we consider this is an initial paper examining the methods and showing they have promise. As discussed in the final section, there are other issues such as investigation of other geographical areas that also need an in-depth further tudy. Following the referee’s suggestion, we have improved the discussion of the validation and training set and established a new subsection 3.2 for this. This topic is also listed in conclusions as an important area for further work. We have added the referee’s suggested reference to the new subsection 3.2.
We thank all the referees for their excellent comments which have improved our paper significantly. The paper has been reformatted in latex and re-organized and so there are many changes
We have replaced all the requested figures. The text describing marked earthquakes is removed from figures and placed in Table 1
Reviewer 3 Report
Please finalize the sentence in line 567.
Please make larger the fonts in Figures 5, 12, 16,17,18,19. They are not readable in the present form. Some of the above figures (12-19) are blurred. Please improve them.
Author Response
We have replaced all the requested figures. The text describing marked earthquakes is removed from figures and placed in Table 1