A Safe Admittance Boundary Algorithm for Rehabilitation Robot Based on Space Classification Model
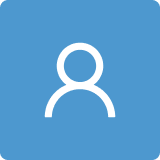
Round 1
Reviewer 1 Report
The authors developed a safe admittance boundary algorithm for a rehabilitation robot.
This research could be a very good contribution to this field of study.
One point, would the authors mention the clinical outcomes that this study will bring to the field?
Author Response
Dear Reviewers:
Thank you for your letter and for the reviewers’ comments concerning our manuscript entitled “A Safe Admittance Boundary Algorithm for Rehabilitation Robot Based on Space Classification Model”. (ID: applsci-2333755). Those comments are all valuable and very helpful for revising and improving our paper, as well as the important guiding significance to our researches. We have studied comments carefully and have made correction which we hope meet with approval. Revised portion are marked in red in the paper(see attachment 1). The main corrections in the paper and the responds to the reviewer’s comments are as flowing:
Responds to the reviewer’s comments:
Reviewer #1:
- Response to comment: Would the authors mention the clinical outcomes that this study will bring to the field?
Response: It’s a great honor to receive your recognition. Special thanks to you for your good comments. In response to the questions you mentioned: In the experiment, we collected the six-dimensional force data of wrist output of 20 groups of people in the actual dynamic motion process (see 3.1.2 and figure12 for details), and verified the algorithm in the simulation environment of twinning by using these actual clinical data, and achieved good results (see table2 for details). It is proved that the algorithm has good performance.
We tried our best to improve the manuscript and made some changes in the manuscript. These changes will not influence the content and framework of the paper. And here we did not list the changes but marked in red in revised paper.
We appreciate for Editors/Reviewers’ warm work earnestly, and hope that the correction will meet with approval.
Once again, thank you very much for your comments and suggestions.
Thank you and best regards.
Yours sincerely,
Corresponding author: Yong Tao
E-mail: [email protected]
Author Response File: Author Response.docx
Reviewer 2 Report
The paper proposes a method to model the environment in which a rehabilitation robot must operate to design virtual limitations to the employed admittance control, ensuring collision avoidance and safety in its operation.
Comments:
1. consider citing [1] that provides an overview of upper limbs rehabilitation exoskeletons control;
2. the novelty of the proposed paper is not clear. Please highlight it w.r.t. state of the art with bullets to make it more clear;
3. Figure 1 needs a better caption describing it;
4. what's r in (3)?
5. the block M^-1=(Fe - B dot_x_e - K x_e) in Figure 6 seems wrong. The = sign shouldn't be there;
6. the admittance model in (7) is just considering traslational DoF, is this correct? How rotations are managed?
7. it is not clear how the stiffness is regulated in (7) and how stability is preserved;
8. in (7) the human dynamics is not modeled. Why? Other approaches (e.g., [2]) are modeling the human-robot interaction and developing controllers with stability guarantees;
9. is there a video of the performed experiments?
10. the proposed strategy is not evaluated w.r.t. the human's perception. This analysis (e.g., the one in [3]) has to be included;
11. how the control gains are tuned? Would it be possible to apply a preference-based optimization as in [4] to maximize the control performance w.r.t. the human's feedback?
12. no comparison w.r.t. the state-of-the-art methods is provided;
13. check for typos.
To conclude, the proposed paper doesn't seem to be novel. In addition, the control design is not novel and it is not clear how the stiffness is adapted and how the stability of the controller is guaranteed. The human dynamics is not considered and this is not justified in the paper. Finally, the experimental validation lacks of the evaluation of the performance of the approach w.r.t. the user perception point of view. The paper needs a very major revision.
[1] Dalla Gasperina, Stefano, et al. "Review on patient-cooperative control strategies for upper-limb rehabilitation exoskeletons." Frontiers in Robotics and AI 8 (2021): 745018.
[2] Roveda, Loris, et al. "Q-Learning-based model predictive variable impedance control for physical human-robot collaboration." Artificial Intelligence 312 (2022): 103771.
Author Response
Dear Reviewers:
Thank you for your letter and for the reviewers’ comments concerning our manuscript entitled “A Safe Admittance Boundary Algorithm for Rehabilitation Robot Based on Space Classification Model”. (ID: applsci-2333755). Those comments are all valuable and very helpful for revising and improving our paper, as well as the important guiding significance to our researches. We have studied comments carefully and have made correction which we hope meet with approval. Revised portion are marked in red in the paper(see attachment 1). The main corrections in the paper and the responds to the reviewer’s comments are as flowing:
Responds to the reviewer’s comments:
Reviewer #2:
- Response to comment: consider citing [1] that provides an overview of upper limbs rehabilitation exoskeletons control;
Response: Thank you for providing this review article, which has very profound insights into effective training methods and hardware implementation of rehabilitation, and gives us a more comprehensive understanding on the three-level control strategy of the upper limb rehabilitation robot. It is greatly inspired for our work, and we have cited this article (see [11] in the manuscript).
- 2. Response to comment: the novelty of the proposed paper is not clear. Please highlight it w.r.t. state of the art with bullets to make it more clear;
Response: Thank you very much for your suggestion. We have highlighted the innovation points of this article in paragraph 8 of 1. Introduction.
- 3. Response to comment: Figure 1 needs a better caption describing it;
Response: Thanks for your suggestions, we have redescribed Figure 1 in the article.
- 4. Response to comment: what's r in (3)?
Response: Thank you for your question. r in (3) and figure 3 mainly represents the length of voxel grid side length constructed for the first and second point clouds based on octree structure. It is highlighted in the second line of paragraph 4 of 2.2.
- 5. Response to comment: the block M^-1=(Fe - B dot_x_e - K x_e) in Figure 6 seems wrong. The = sign shouldn't be there;
Response: Thank you very much for your reminding. We have modified the formula in Figure 6.
- 6. Response to comment: the admittance model in (7) is just considering translational DoF, is this correct? How rotations are managed?
Response: The problem you mentioned is very professional. In this work, we mainly built an algorithm model based on the isopdensification of point cloud and the nearest neighbor search algorithm. One advantage of incorporating the variable stiffness admittance strategy into this model is that it can carry out reasonable morphological restrictions on the workspace of the DoF at the end of the robot. The DoF of rotation you mentioned may not be the main problem we are dealing with in our current work. However, your proposal has inspired us a lot. We will add restrictions on the freedom of rotation into our work in the future. Thank you very much.
- 7. Response to comment: it is not clear how the stiffness is regulated in (7) and how stability is preserved;
Response: The problem you mentioned is very important. The stiffness in (7) is matched based on the density obtained in the space classification model (see paragraph 4 of 2.4 for details), and the specific value is mainly dependent on empirical parameters at present. Stability is a very core index of the controller, and the core work of this algorithm is mainly in the strategy layer. For the spatial classification model, we have carried out space verification experiments (see 3.2.1), and the results of experimental parameters such as space definition accuracy in the experiment (see table1) could prove the stability of our algorithm.
- Response to comment: in (7) the human dynamics is not modeled. Why? Other approaches (e.g., [2]) are modeling the human-robot interaction and developing controllers with stability guarantees;
Response: Thanks for your question and suggestion. The method in [2] introduces human dynamic into admittance control, which can improve the accuracy and adaptability of admittance control to a large extent. In practical applications, we consider the similar method with [2]: we consider the dynamics of the human body and use static compensation for admittance parameters after parameter calibration. Before modification, considering the spatial classification model at the strategy layer as the core of this paper, in order to reflect the universality of the method in different human-computer interaction scenarios, it was not expanded in (7). We attach great importance to your proposal. We have explained it again in the article (see paragraph 4 of 2.4 for details) and quoted [2] (see reference [10]).
- Response to comment: is there a video of the performed experiments?
Response: We are very glad that you are interested in our research and we can provide a video of the actual algorithm verification (see Attachment 2 for details).
- Response to comment: the proposed strategy is not evaluated w.r.t. the human's perception. This analysis (e.g., the one in [3]) has to be included;
Response: Thank you for your question. In the experiment, we actually collected the six-dimensional force data of wrist output of 20 groups of real people in the actual dynamic motion process (see 3.1.2 and figure12 for details), and verified the algorithm in the simulation environment of twinning by using these actual clinical data, and achieved good results (see table2 for details). It is proved that the algorithm has good performance. For the feedback of human beings, we added the comparison of human kinesthetic parameters in the fourth line of paragraph 5 of the manuscript 3.2.2, proving that the security algorithm has a good feedback effect.
- Response to comment: how the control gains are tuned? Would it be possible to apply a preference-based optimization as in [4] to maximize the control performance w.r.t. the human's feedback?
Response: Thank you very much for your suggestions. The control gains are tuned by fuzzy adaptive control. We have applied the method [4] in the human-computer interaction system and achieved good results. We have cited it in the manuscript (see reference[33]).
- Response to comment: no comparison w.r.t. the state-of-the-art methods is provided;
Response: Thank you for your professional suggestion. We hope to compare the existing most advanced algorithms and prove the advancement of our method. However, due to the particularity of equipment and application scenarios, we have not found a suitable and public similar algorithm for comparison at present. We attach great importance to your suggestion. Based on our article, we add an experimental parameter comparison to demonstrate the effectiveness of this algorithm, which should better reflect the performance and advancement of the algorithm (see the fourth line of paragraph 5 of 3.2.2 for details).
- Response to comment: check for typos.
Response: Thanks for your advice, we have corrected the spelling mistake in the article.
- Response to comment: To conclude, the proposed paper doesn't seem to be novel. In addition, the control design is not novel and it is not clear how the stiffness is adapted and how the stability of the controller is guaranteed. The human dynamics is not considered and this is not justified in the paper. Finally, the experimental validation lacks of the evaluation of the performance of the approach w.r.t. the user perception point of view. The paper needs a very major revision.
Response: Thank you very much for your suggestions on the paper. We have highlighted the innovation points of the paper and made a new reference to the controller. We have also explained the adaptation of stiffness. Human dynamics have been added in our work. For the experience of users in the experiment, parameters in reference are also added in the experiment for comparison.
We tried our best to improve the manuscript and made some changes in the manuscript. These changes will not influence the content and framework of the paper. And here we did not list the changes but marked in red in revised paper.
We appreciate for Editors/Reviewers’ warm work earnestly, and hope that the correction will meet with approval.
Once again, thank you very much for your comments and suggestions.
Thank you and best regards.
Yours sincerely,
Corresponding author: Yong Tao
E-mail: [email protected]
Author Response File: Author Response.docx
Round 2
Reviewer 2 Report
The paper has been reviewed and can be now accepted.