Evaluation of Metamorphic Testing for Edge Detection in MRI Brain Diagnostics
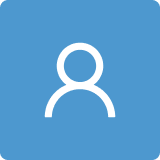
Round 1
Reviewer 1 Report
The paper titled “Evaluation of Metamorphic Testing for Edge Detection in MRI Brain Diagnostics” has applied metamorphic testing to edge detection algorithms used in MRI brain diagnostics and evaluated the fault detection effectiveness of four metamorphic relations used in metamorphic testing. However, the authors may address the comments given below:
11. The authors should develop a table of state-of-the-art methods from some reputed Science Citation Indexed journals mentioning the methods, advantages, and disadvantages to make clear the research gap.
22. There are many recent techniques of deep learning-based edge detection and segmentation techniques for detecting brain tumors, stroke lesions, white matter, gray matter, CSF, etc. The author should mention them in the literature review.
T3. The authors may cite the following papers and should mention why they are not considering these most recent machine learning-based algorithms for testing:
(a) “MhURI: A Supervised Segmentation Approach to Leverage Salient Brain Tissues in Magnetic Resonance Images”, Computer Methods and Programs in Biomedicine, Elsevier, Vol. 200, pp. 105841, 2021.
(b) "A Dense U-Net Architecture for Multiple Sclerosis Lesion Segmentation". IEEE Region 10 Conference (TENCON), IEEE, October 17-20, 2019.
(c) “Edge detection algorithm of cancer image based on deep learning”, Bioengineered, Taylor & Francis, pp. 693-707, 2020
34. Is it possible to apply Metamorphic Testing to evaluate more recent deep learning-based edge detection algorithms?
45. Novelty of the work is not clear
56. Conclusion of the work should be strengthened with numerical results obtained during testing.
Author Response
please see the attachment
Author Response File: Author Response.docx
Reviewer 2 Report
Not clear from the abstract the main objective of this work. Several strategies exist to test the robustness of image processing algorithms:
- the algorithm is developed on a training set
- the algorithm is evaluated on a validation set
- performance is evaluated on one or more external test sets.
If the performance of the system is satisfactory and there is no performance degradation between training and testing (overfitting), then the tool can be considered reliable. In addition, nowadays several AI explainability tools are emerging.
Author Response
please see the attachment
Author Response File: Author Response.docx
Round 2
Reviewer 1 Report
1. The image processing community is rapidly moving towards machine learning-based image processing applications. Testing and validation of machine learning-based algorithms are now stringent requirements and the scope of work in the field of software testing and validation. Hence, metamorphic testing of some recent works on Machine learning/Deep-Learning-driven image processing systems should be described and compared with the proposed system.
Author Response
Please see the attachment
Author Response File: Author Response.docx
Reviewer 2 Report
The revised version is not improved. Novelty very limited. Nowadays deep learning has become the methodology of choice for medical imaging tasks (classification, detection, segmentation, etc.)
Author Response
Please see the attachment
Author Response File: Author Response.docx
Round 3
Reviewer 2 Report
The revised version is clear and more focused.