Data Augmentation by an Additional Self-Supervised CycleGAN-Based for Shadowed Pavement Detection
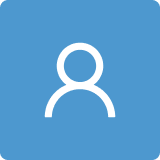
Round 1
Reviewer 1 Report
Following questions should be settled:
1. This paper mentioned that for shadow texture images, it can be generally considered as the difference between the semantic information of image in Y domain and that of image in X domain. Is the difference here a simple difference relation? It is mentioned in this paper that the image semantics in Y domain is equivalent to the sum of image semantics and shadow semantics in X domain, but this relation is not a simple additive relation, and the relation here needs to be explained.
2. The function expressions in Sections 2.3.2 and 2.3.3 should include an explanation of the parameters.
3. The title name of Section 2.3.4 does not correspond to the loss name mentioned above.
4. It is recommended that the title of section 2.3.5 be changed to Overall Loss Function.
5. What is the basis for setting the weight parameter in Section 2.3.5?
6. In Figure 5, the training times only reached 50 epochs, and no control experiment with higher training times was conducted. Why was it determined that the model generation effect would converge if the training times reached 50 times?
7. In Figure 6, why the new CycleGAN with a texture self-supervised loss function can achieve better generation effect in semantic generation tasks?
8. The test description section for Figure 9 is missing.
9. In Figure 9, why is the segmentation effect of a small number of data still not obvious or even not as good as before the Segmentation? Please explain why?
Author Response
Please see the attachment.
Author Response File: Author Response.docx
Reviewer 2 Report
The submitted manuscript describes a very hot and interesting topic. The authors have proposed an improved shadow generation network, named Texture Self-Supervised CycleGAN (CycleGAN-TSS), to improve the effect of generation and augment the band of shadowed images of pave-ment cracks. The model is established and evaluated through numerical experiments. The results seem to be effective and feasible. This is well appreciated by this reviewer. However, to further improve the quality of this work, several minor points should be further concerned by the authors:
a. The authors are advised to carefully check throughout this manuscript to avoid typos and improve english writing.
b. The first and last sentences of the Abstract should be revised. Moreover, some figures should be further improved, e.g., Figures 2 and 8.
c. In Section 1, some very recent and related data-driven-based papers on image detection and materials design should be briefly mentioned and cited. Such as: Machine learning-enabled high-entropy alloy discovery, Science; Structural symmetry recognition in planar structures using convolutional neural networks, Engineering Structures; Machine learning applied to the design and inspection of reinforced concrete bridges: Resilient methods and emerging applications, Structures.
d. What about the accuracy of the proposed method?
e. Can the method be used/extended to detect 3D objections, such as depression and uplifts of a pavement?
Author Response
Please see the attachment.
Author Response File: Author Response.docx
Reviewer 3 Report
The paper is presented very well. The topic that is choose is intresting and current. I have some suggestion to be more clear and usefull the results from the experiment can be more detailed. Figure 8 can be more clear.
Author Response
Please see the attachment.
Author Response File: Author Response.docx
Reviewer 4 Report
The authors present a paper for the detection of crack pavements using deep learning methods. In this paper, the Cycle Gan method has been selected . Below my review comments for your paper:
1) Elaborate more about paired and unpaired datasets
2) Justify your selection of CycleGan in your problem
3) Explain about Cycle consistency
4) For the network structure Fig.4 you need to add the missing information about the different layers
5) Explain the difference between discriminative and generative algorithms
6) Specify if we have forward or backward cycle consistency
7) Identity loss is missing from Fig.2
8) Explain how selected the weights?
9) Missing the aiming to solve the full objective function.
Reference list is fine.
Author Response
Please see the attachment.
Author Response File: Author Response.docx
Round 2
Reviewer 1 Report
Raised questions were settled in the revised manuscript. This paper is now ready to be accepted for publication.