Real Time Implementation of Learning-Forgetting Models for Cycle Time Predictions of Manual Assembly Tasks after a Break
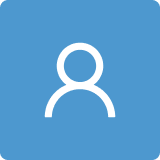
Round 1
Reviewer 1 Report
This scientific paper is very interesting and described results are interesting even more. Problem of this paper is that the overall structure and descriptionof methods is not in sufficient level.
Description of achivied results and used mehtods needs to be re-writed.
Author Response
Please see the attachment.
Author Response File: Author Response.pdf
Reviewer 2 Report
The paper approaches an interesting topic.
In this study, different existing models were compared in order to predict the cycle time after a break. As these models are not created for a real-time prediction purpose, some adaptations are presented in order to improve the robustness and efficiency of the models.
The paper comprises a number of studies on the subject of interest.
Methodology needs to be completed with more information:
- more details about the structure of the research and sampling on different models - why learning forgetting models, how you chose the models, what criteria were used, how you checked them - it must be described in such a way that the study can be repeated.
- the choice of the methodological method needs to be justified: why the real-time implementation? What are the purposes, advantages of this method and why is it appropriate for the present research. Justify with arguments from the literature.
The results section can also be improved:
- start the section with an introductory sentence/paragraph in which to remind what is that you have studied and what is that you are going to present in this section.
What are the limitations of the study? Please, this issue into Conclusion.
Author Response
Please see the attachment.
Author Response File: Author Response.pdf
Reviewer 3 Report
The theoretical explanation and validation of multiple approaches of learning-forgetting model of manual assembly tasks is well presented allowing the reader to smoothly undertand the concept. The validation is scientific (comparison of multiple methods, statistical significance) which provides to the reader trust in the published results.
One point I had in mind while reading the paper, is that the models don t take into account the environment of the operator, maybe the period of the year (?)...performing a task in a noisy environement can influence how deep will be the learning of that task by the operator, so how quickly that task will be forgotten. I guess there are a lot of contextual information that could influence the prediction of the cylce time. Machine learning could play an interresting role here by augmenting the dataset provided by the Arkite device with contextual data.
The Arkite device seems to provide a lot of data that can be analyze with machine larning without relying on a specific model. a pure machine learning approach, if there is enough data, could be a next step to investigate.
small other comments:
- abstract: define PID and MLFCM
- line 91: ',' misplaced
- section 4: maybe a table with additional detail about the data recorded, and some stats, would help the reader to extrapolate to other solution like machine learning
Author Response
Please see the attachment.
Author Response File: Author Response.pdf
Round 2
Reviewer 1 Report
I'm OK with authors changes.