Discovering Low-Dimensional Descriptions of Multineuronal Dependencies
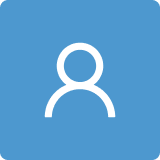
Round 1
Reviewer 1 Report
Comments for author File: Comments.pdf
Author Response
We thank the reviewer for the positive assessment of our work and the insightful comments.
> Although explained in the introduction, the results section should start explaining the goal of your factorization. It took me a while for understanding on what (and why) you were applying WNMF. Similarly, the corresponding method section (2.5) is generic: what are X,W and H in this study? As a reader I was initially lost when reading the results, and going to the methods was of little help. The authors should add a few additional explanations at the beginning of the results section, and maybe update the methods accordingly.
We thank the reviewer for raising this point. We have revised the text in the introduction, methods, and results section in order for the goal of our analysis to be clearer. Briefly, our aim was to characterize neural dependencies with a non-parametric C-vine copula-based method. Following this approach, the non-parametric copula densities would be further decomposed into a parts -based low dimensional representation with WNMF. We made more explicit mentions to the fact that it is copula densities that we are interested in estimating and then giving as input to WNMF for dimensionality reduction. Specifically, we mention it in the introduction section, page 2, lines 78 and 92 as well as page 3, lines 102 and 117-118. We also included explicit mentions of copula densities in the methods section 2.1, page 4, lines 145-146 and methods section 2.5, page 6, line 205 where we also point to Figure1C. Lastly, we now mention copula densities in the results section page 7, lines 272-275. Regarding the meaning of W and H, in the methods section 2.5, page 6, lines 208-209 we make a more explicit reference to W and H as the neuron pair coefficients and the copula modules accordingly, which are the terms we consistently use in the results section. Moreover, in the methods section 2.5, page 6 lines 213-217, we added an explanation of the rationale behind why we use WNMF. We hope that these additions improve the flow of the manuscript and contribute to an easier and conducive to understanding reading.
> If I'm not mistaken when you applied WNMF on real data, you used non-parametric copulas, but on the example on synthetic data (teacher student) you used parametric copulas like Frank, Clayton and rotations. Is there a reason for this?
Our WNMF analysis on neural recordings was indeed based on non-parametric copulas. Parametric copulas in the synthetic datasets were employed to generate samples from copulas with known dependence structures to serve as ground truth for validating WNMF and compare it to regular NMF in different conditions such that we could demonstrate where WNMF outperforms NMF.
> One particular advantage of using copulas is that it allows to mix variables of different nature, like spike counts with running velocity, position on a track or even brain states. Most other statistical tools will struggle in combining them, yet copula can do it, and authors have already shown that this is doable (Kudryashova et al PCB 2022). Yet in this current paper the authors focus only on neurons, somehow narrowing the scope of their method. I suggest this can be at least discussed with some details
Copulas do indeed allow for mixing variables with different statistics. However, our focus on this paper was different, i.e. primarily to leverage low dimensional structure in population-wide multineuronal dependencies and to show how one can use WNMF to achieve a more easily interpretable representation of these dependence structures. We didn’t focus on showing that behavioral variables can also have complicated dependencies with neural variables, even though this is most likely the case and can be the subject of future research directions with non-parametric copula-based approaches. We included a brief discussion of the above along with a few other limitations in the discussion section, page 13, lines 438-453
> If it is true that copulas have not been used much in computational neuroscience -LL61-63, pag 2-, there are a few relevant papers that should be cited (example Berkes NeurIPS 2008), including some from the authors themselves that should be reminded after these lines.
We have now included additional citations to a few more relevant papers in the introduction section, page 2, lines 62-63, where we also slightly edited the text to better convey that there have been a few studies by different groups of authors but copulas have received much less attention in neuroscience compared to economics.
> Is there a reason why a c-vine has been chosen, instead of other vine copulas?
The general class of regular vines is a very flexible model class which allows for numerous dependency structures. It is desirable to limit this flexibility in order to make the model identifiable. The C-vine or canonical vine accomplishes this by making the dependency structure more rigid. It is a special case of a regular vine, consisting of a hierarchy of trees where each tree has a node that serves as a connection hub to all other nodes. The canonical vine is the most commonly used vine structure. Moreover, this structure matches functional connectivity patterns in neural recordings very well since typically the activity of a few neurons shapes the activity of many other neurons.
We clarified the reasons for choosing the C-vine in the manuscript (page 5, lines 162-165).
> LL188, page 5, a reference is missing
Fixed. Due to changes in the text it was moved to line 193.
> LL421 - 433, page 13: Either this paragraph is really badly written, or this text was meant to be erased before submission.
We apologise for this mistake and thank reviewer #1 for noticing it. It was indeed a part of text from a previous version of the manuscript that was meant to be erased before submission. It has been erased now.
Reviewer 2 Report
Summarize the paper: The paper focuses on distilling dependencies between neurons. The input is neuron spike count histograms. The inverse cumulative probabilities of single neuron spike count histograms were used to build weight matrices for each bivariate copula. Then, they adopt a framework called weighted non-negative matrix factorization (WNMF) to extract the copula densities. The proposed method is evaluated on both synthetic and practical data.
Main weaknesses:
- The readers likely find it difficult to follow Section 2 due to the lack of problem clarification such as the problem definition, input (what is the data?), output, and variable definition.
- The paper lacks a discussion on the use of the final output. I can understand the technical details of the paper, however, it is unclear to the readers how NMF neuron pairs factors and NMF copula modules will be used in subsequent analysis or downstream tasks.
Strengths:
- Interesting problem.
- Comprehensive experiments.
Questions:
- Using C-vine seems ad-hoc. The authors should give further justification for this choice of method: why C-vine and not any other vine copula? Is there any problem-specific characteristic that makes C-vine an optimal modeling choice?
- Can you provide the additional human-interpretable meaning of NMF neuron pair factors and NMF copula factors in Figure 1?
- My understanding is that the copula should have values in [0,1]. Thus, the NMF should be a constrained problem, in which we need to constrain the copula modules to admit values in [0,1]. Can the authors please explain why this constraint is not imposed in Section 2.5?
Minor problems:
- Line 13: “Out findings” -> “Our findings”
- Line 21: “resentations” -> “representations”
- Line 188: Missing links [?]
Some minor editing will make the paper easier to read.
Author Response
We thank the reviewer for the positive assessment of our work and the insightful comments.
> The readers likely find it difficult to follow Section 2 due to the lack of problem clarification such as the problem definition, input (what is the data?), output, and variable definition.
We thank the reviewer for raising this point. We have revised the text in the introduction, methods, and results section in order for the goal of our analysis to be clearer. Briefly, our aim was to characterize neural dependencies with a non-parametric C-vine copula-based method. Following this approach, the non-parametric copula densities would be further decomposed into a parts -based low dimensional representation with WNMF. We made more explicit mentions to the fact that it is copula densities that we are interested in estimating and then giving as input to WNMF for dimensionality reduction. Specifically, we mention it in the introduction section, page 2, lines 78 and 92 as well as page 3, lines 102 and 117-118. We also included explicit mentions of copula densities in the methods section 2.1, page 4, lines 145-146 and methods section 2.5, page 6, line 205 where we also point to Figure 1C. Lastly, we now mention copula densities in the results section page 7, lines 272-275. Regarding the meaning of W and H, in the methods section 2.5, page 6, lines 208-209 we make a more explicit reference to W and H as the neuron pair coefficients and the copula modules accordingly, which are the terms we consistently use in the results section. Moreover, in the methods section 2.5, page 6, lines 213-217, we added an explanation of the rationale behind why we use WNMF.
We hope that these additions improve the flow of the manuscript and contribute to an easier and conducive to understanding reading.
> The paper lacks a discussion on the use of the final output. I can understand the technical details of the paper, however, it is unclear to the readers how NMF neuron pairs factors and NMF copula modules will be used in subsequent analysis or downstream tasks.
We thank the reviewer for raising this point. We expanded the discussion to include a few points about potential subsequent analyses involving WNMF copula modules along with a few limitations in page 13, lines 438-453
> Using C-vine seems ad-hoc. The authors should give further justification for this choice of method: why C-vine and not any other vine copula? Is there any problem-specific characteristic that makes C-vine an optimal modeling choice?
The general class of regular vines is a very flexible model class which allows for numerous dependency structures. It is desirable to limit this flexibility in order to make the model identifiable. The C-vine or canonical vine accomplishes this by making the dependency structure more rigid. It is a special case of a regular vine, consisting of a hierarchy of trees where each tree has a node that serves as a connection hub to all other nodes. The canonical vine is the most commonly used vine structure. Moreover, this structure matches functional connectivity patterns in neural recordings very well since typically the activity of a few neurons shapes the activity of many other neurons.
We clarified the reasons for choosing the C-vine in the manuscript (page 5, lines 162-165).
> Can you provide the additional human-interpretable meaning of NMF neuron pair factors and NMF copula factors in Figure 1?
We edited the text in methods section 2.5 page 6 lines 205-211 to provide a slightly more in depth explanation of the neuron pair coefficients “W” and the copula modules “H” produced by NMF or WNMF. Also, we provide the interpretation for W and H in the case of analyzing neural recordings, ie that H are interaction patterns shared across the neuronal population and W is the degree to which specific neuron pairs, as they are defined by the C-vine are displaying one or a combination of these interaction patterns (methods section 2.5, page 6, lines 213-217).
> My understanding is that the copula should have values in [0,1]. Thus, the NMF should be a constrained problem, in which we need to constrain the copula modules to admit values in [0,1]. Can the authors please explain why this constraint is not imposed in Section 2.5?
The samples in the copula space are indeed constrained in [0,1]. However, the input to WNMF are the copula densities which are non-negative but can be greater than 1. WNMF searches for the neuron pair coefficients “W” and copula density modules “H” that will closely reconstruct the data matrix “X” which corresponds to the vine copula densities.
> Comments on the Quality of English Language: Some minor editing will make the paper easier to read.
We polished the language and clarified the text throughout.
> Line 13: “Out findings” -> “Our findings”
Fixed.
> Line 21: “resentations” -> “representations”
This was due to a line break which caused the word “representations” to be split into rep-resentation between two lines.
> Line 188: Missing links [?]
Fixed. Due to changes in the text it was moved to line 193.
Reviewer 3 Report
Summary
This paper describes a copula-based method for summarizing multi-neuronal interactions via a low number of factors. The method builds on the authors’ previous work using normalizing flows to fit bivariate copulas as part of a C-vine construction. The C-vine construction factorizes the full multivariate dependency into pairwise copulas, which are learned via normalizing flows with an independence test to only fit pairs of distributions that are not independent under a hypothesis test. An extension of the method proposed in this paper is a weighted nonnegative matrix factorization to decompose the set of all pairwise copulas into a product of per-copula weights and a low number of copula factors.
The method is applied in both simulations and to neural recordings. In simulations, the authors motivate their weighted formulation of the nonnegative matrix factorization and verify its performance. Then, the method is applied to model neural interactions from mouse V1 cortex and monkey motor cortices. In both applications the approach identifies a low number of factors that capture pairwise variability in the copula distributions.
Overall, the proposed method appears important to identifying structure in neural population responses and shows promising results in the simulated experiments. However, I have some questions about the methods and experimental applications that I think should be addressed before I can recommend the paper for acceptance. Please see the comments below.
Major Comments
-
Modeling the bivariate dependencies in the C-vine construction with normalizing flows is an interesting approach that appears to work well. Additionally, the proposed factorization method of copula densities appears to provide meaningful results in the simulated data. However, I have questions about the joint method that combines these approaches for modeling neural data.
-
First, it is not clear to me that it makes sense to jointly factorize all of the dependent copulas that are obtained via the pairwise vine construction. In particular, my understanding is that the copulas in this factorization are obtained from distributions that condition on different numbers of variables (neurons). Why does it make sense to find low dimensional structure across this set of distributions with varying conditioning variables? More intuition, explanation, and results would be helpful for convincing me of the utility of the coefficients and factors in this case.
-
Does the ordering of the vine have any effect on estimation of the factors or coefficients?
-
The simulated example (Fig 3) provides nice empirical support for the proposed methods. I have a few comments regarding the presentation of this experiment.
-
The authors state that in Line 291 that the optimal number of factors (6) was not clearly indicated by the NMF validation curve. Is this true? It looks like NMF identifies 6 as the lowest validated error.
-
It would be helpful to reorganize the plots in 3A. I suggest having two columns for train and val, and on each panel plot the results for WNMF and NMF in separate colors. This will make sure the methods are plotted on the same axes and will help with direct comparisons between them.
-
Likewise, I suggest making the y axes the same for 3B. It isn’t obvious at first that WNMF is recovering the true factors by orders of magnitude, which is impressive!
-
To provide more intuition about the weighting matrix V, can you show what it looks like in this simulation?
-
I appreciate the application of the proposed methods on two different neural dataset. With some changes and additional analyses, I think these analyses can provide strong empirical support for the method’s utility on real neural data.
-
In the coefficient plots on Fig.4-5, are the scale of the WNMF coefficients comparable across factors? Or does different scaling in the copula factors make it hard to directly compare WNMF coefficient weights? If it makes sense to plot the different factor coefficients on the same y-axes scale, I suggest doing that for additional clarity and insight.
-
Additionally it would be very helpful to show examples of individual copulas reconstructed from the four factors, to aid with interpretation of the coefficients on the factors.
-
Would an alternative metric, like e.g. fraction of variance explained by weight_i * factor i for copula j, be interesting to look at alongside the factor coefficients? I’m wondering if such a metric would convey information that the factor coefficients do not by themselves.
-
In both the mouse and monkey neural data analyses, currently I am convinced that one can factorize the copula dependencies in this way but it is not clear how to use this to get insight into how the neural responses serve the task at hand. I think the experimental support for this method would be improved if the authors can relate the resulting copulas, factors, and coefficients to the neural code during virtual navigation or reaching.
Minor Comments
-
The clarity of the methods could be improved with some additional details, which would also help with evaluation.
-
The important equations of the sequential estimation procedure described in Sec. 2.4 should be included.
-
In the WNMF section, please include the equations describing how V is computed. Additionally, it would be helpful to see what V looks like in the examples.
-
The authors cite additional papers on weighted nonnegative matrix factorization [42,43]. It would be great to explain how the proposed method relates to these papers and other WNMF approaches in the literature.
-
While the L1 regularization in the WNMF sounds helpful, it is important to note that this setup will not generally introduce sparsity in the solution without a thresholding operator update.
Typos
Line 115 - “.. the aforementioned..” - missing a word after this?
Section 2.1 -> “as according Sklar’s theorem” -> “as according to Sklar’s theorem”
Line 188 - Missing ref?
Final paragraph - it appears the final paragraph with a list of potential discussion points was erroneously included.
N/A
Author Response
Dear members of the Entropy Journal Editorial Board,
Thank you for providing us with an opportunity to revise our Manuscript entitled “Discovering low-dimensional descriptions of multineuronal dependencies” by L. Mitskopoulos and A. Onken. We now wish to submit our revision of this manuscript for further consideration.
In this revision, we addressed the reviewer comments point by point. We list these points below. We are grateful to the reviewers for their insightful comments, which helped us significantly improve the manuscript.
Thank you very much for your consideration.
Sincerely yours,
Lazaros Mitskopoulos on behalf of all authors
The figures that we refer to in this response are included in the accompanying pdf file entitled "rebuttal rv3 Mitskopoulos.pdf"
We thank the reviewer for the positive assessment of our work and the insightful comments.
> 1a. First, it is not clear to me that it makes sense to jointly factorize all of the dependent copulas that are obtained via the pairwise vine construction. In particular, my understanding is that the copulas in this factorization are obtained from distributions that condition on different numbers of variables (neurons). Why does it make sense to find low dimensional structure across this set of distributions with varying conditioning variables? More intuition, explanation, and results would be helpful for convincing me of the utility of the coefficients and factors in this case.
The copulas in the C-vine can be jointly factorized by virtue of the fact that the dependencies occurring in the vine tree can share similar features. We inspected copula densities from different trees and found that despite conditioning sets being different, there is shared structure, as demonstrated with a few illustrative examples in the figure below. These examples show copula densities that follow the positive correlation path and concentrate probability mass in the bottom left and top right corners to different extents. Since our goal is to summarize multivariate dependence structures in neural ensembles, it is a reasonable suggestion to conduct a joint decomposition. Note also that the linear combinations of copula factors explained more than 95% of most copulas in the different trees (c.f. answer to question 3c) indicating that a single factorization across trees is indeed appropriate.
Furthermore, the information on the conditioning sets and the specific tree one finds dependencies of a certain kind is still accessible and we added an additional figure (Figure 5) in page 12 in the main text which illustrates how the coefficients for different WNMF copula modules reveal clustering across trees such that there is a divide between superficial and deeper trees regarding the copula modules that are dominant.
> 1b. Does the ordering of the vine have any effect on estimation of the factors or coefficients?
The ordering of the vine does have a significant effect on the types of dependence structures one can find in a multivariate distribution when using vine copula approaches. Finding the optimal order is a challenging problem but it is common to rely on heuristics like the one we used in this study which has also been employed before (Onken et al., 2016. Kudryashova et al., 2020) and relies on the sum of absolute Kendall’s tau values for each neuron in relation to all the other neurons. The neurons can be ranked starting from the one with the largest sum and ending at the one with the lowest. We thank the reviewer for raising this point as we did omit to mention it explicitly in the text. We now added a few sentences to that end on page 5, lines 190-193. It’s a nice heuristic as in C-vines we expect the first variable to be playing the role of a hub. Therefore, in our applications it is crucial which neuron is selected first for the kind of dependence structures you can find. The decomposition can be quite different with a different ordering. In the figure below, we illustrate such a case where we chose a random ranking of the neurons from mouse V1. This ended up in fewer independent copulas (546 as opposed to 811) and the WNMF coefficients are not grouped similarly to the decomposition we show in Figure 4.
>2a. The authors state that in Line 291 that the optimal number of factors (6) was not clearly indicated by the NMF validation curve. Is this true? It looks like NMF identifies 6 as the lowest validated error.
We indeed found that in the case of artificial data generated by 6 factors, NMF yielded inconclusive results. If one looks closely at the validation error curve for the bottom right panel at Fig 3A, the values for 4, 5 and 6 factors look quite similar. Obviously, one could still take the minimum across these 3 configurations, but the point we were aiming to make with this example is that the inflection point where the validation error starts increasing is much more obvious for WNMF than it is for NMF. Therefore, the optimal number of factors is easier to select with WNMF.
>2b. It would be helpful to reorganize the plots in 3A. I suggest having two columns for train and val, and on each panel plot the results for WNMF and NMF in separate colors. This will make sure the methods are plotted on the same axes and will help with direct comparisons between them.
We sincerely appreciate this suggestion but upon attempting to reorganize the plots in 3A such that there are separate columns for train and validation error, we found that this organization is actually less helpful in making comparisons between the methods. In the left panel of the figure below, we illustrate how plotting the validation error curves for WNMF and NMF in the case of 6 factors makes the NMF curve look much flatter than what it is if one plots it together with the train error. While it certainly is interesting that the validation error is considerably lower for WNMF, the illustration in the figure below would leave the impression that the NMF validation error reaches a plateau after 3 or 4 factors which is not accurate.
> 2c. Likewise, I suggest making the y axes the same for 3B. It isn’t obvious at first that WNMF is recovering the true factors by orders of magnitude, which is impressive!
We updated Figure 3 by reorganizing the panels in 3B such that WNMF and NMF in each case now share the same y axis. For illustration purposes, since the errors between the methods were 2 orders of magnitude apart, we used a logarithmic scale for y axis.
>2d. To provide more intuition about the weighting matrix V, can you show what it looks like in this simulation?
We illustrate examples of the V matrices for simulated data (A) and for spiking data from neural recordings (B) in the figure below. The colorbar is set to min and max instead of real numbers as the actual values tend to differ across artificial variables and neuron pairs. The most significant feature that is shared among all the weight matrices is the higher values on the tail regions and comparatively lower values elsewhere.
> 3a. In the coefficient plots on Fig.4-5, are the scale of the WNMF coefficients comparable across factors? Or does different scaling in the copula factors make it hard to directly compare WNMF coefficient weights? If it makes sense to plot the different factor coefficients on the same y-axes scale, I suggest doing that for additional clarity and insight.
Even though we normalized all the copula densities estimated from NSF such that each of them sums to unity, this is not the case for the WNMF copula modules. These modules have uniform margins which is a fundamental property of copulas but they do not sum to unity and have different scales to one another. This indeed makes it challenging to directly compare WNMF coefficient weights across factors.
>3b. Additionally it would be very helpful to show examples of individual copulas reconstructed from the four factors, to aid with interpretation of the coefficients on the factors.
In the figure below we illustrate representative examples of individual copula densities, the WNMF reconstruction and the specific coefficients that indicate which of the WNMF copula modules are recruited and to what extent for the parts-based representation of the copula densities. For easier reading of the figure we added the WNMF copula modules on the left side such that it is obvious which copula modules are being recruited to reconstruct the copula densities in each of the 4 examples.
>3c. Would an alternative metric, like e.g. fraction of variance explained by weight_i * factor i for copula j, be interesting to look at alongside the factor coefficients? I’m wondering if such a metric would convey information that the factor coefficients do not by themselves.
Unfortunately, fraction of variance explained or R2 for individual factors with respect to specific copulas is not well defined in this case since the WNMF components are not constrained to be orthogonal. Therefore, if one is interested in specific copulas it is only appropriate to calculate these metrics for the linear combination of WNMF copula modules that reconstruct each copula density. In this case we find that the linear combination of WNMF copula modules explains more than 95% of variance in the overwhelming majority (92%) of copulas. However, this indeed does not help with any association of the values of the coefficients with these metrics.
>3d. In both the mouse and monkey neural data analyses, currently I am convinced that one can factorize the copula dependencies in this way but it is not clear how to use this to get insight into how the neural responses serve the task at hand. I think the experimental support for this method would be improved if the authors can relate the resulting copulas, factors, and coefficients to the neural code during virtual navigation or reaching.
While a full assessment of the relation of the resulting copulas, factors and coefficients to the neural code is beyond the scope of this paper, which aims at a low-dimensional description of the neuronal dependence structures, we added a new paragraph to the Discussion section, detailing future directions with respect to the impact of dependence measures on information quantities, behavioral variables and dimensionality of the space of dependence structures (page 14, lines 457-472).
> 4a. The important equations of the sequential estimation procedure described in Sec. 2.4 should be included.
We included the equations plus a brief explanation for the h functions which constitute the tool by which one can estimate conditional margins. (page 6, lines 195-198 )
> 4b. In the WNMF section, please include the equations describing how V is computed. Additionally, it would be helpful to see what V looks like in the examples.
We included the equation plus a brief description of how V is calculated which was indeed needed in pages 6-7, lines 231-242. For an illustration of V both for the artificial data and the data from neural recordings see the figure in our answer on comment 2d
>4c. The authors cite additional papers on weighted nonnegative matrix factorization [42,43]. It would be great to explain how the proposed method relates to these papers and other WNMF approaches in the literature.
We added a few more sentences that explain our proposed approach in more detail while providing more intuition as well in addition to how it differs from similar methods we encountered in the literature in page 7, lines 238-242.
Typos
>Line 115 - “.. the aforementioned..” - missing a word after this?
Fixed. We changed this to “Taking into account the approaches in the aforementioned studies”
>Section 2.1 -> “as according Sklar’s theorem” -> “as according to Sklar’s theorem”
Fixed.
>Line 188 - Missing ref?
Fixed. Due to changes in the text it was moved to line 199.
>Final paragraph - it appears the final paragraph with a list of potential discussion points was erroneously included.
We apologise for this mistake and thank reviewer #3 for noticing it. It was indeed a part of text from a previous version of the manuscript that was meant to be erased before submission. It has been erased now.
Author Response File: Author Response.pdf
Round 2
Reviewer 1 Report
The authors have addressed all my minor concerns. The paper should be accepted
Reviewer 2 Report
The authors have addressed all comments that I posed in the previous round.
Reviewer 3 Report
I thank the authors for their thorough response to my comments and questions. Upon review of the revised manuscript, I am happy to support acceptance for publication.