Language Identification-Based Evaluation of Single Channel Speech Separation of Overlapped Speeches
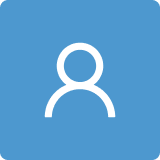
Round 1
Reviewer 1 Report
The authors propose the combination of a source separation algorithm with a language ID system in order to perform language ID of two speakers speaking simultaneously different languages.
The authors appear to be using ready architectures with no or minimal novelty. However, what feels strange to the reviewer is the design of the experiment, which is totally impractical. They create artificial mixture of different language pairs. The source separator is operating regardless of the spoken language. Then, they train a different language ID system to work for the different language pairs. The reviewer sees no point for doing so, since the system should know which language pairs are present in order to select the corresponding network. Instead, a complete language ID system should be used, in order to detect any of the tested languages. In addition, a binary classification system is not interesting, since it can achieve almost perfect classification, as it can be seen in the experiments. A multi-class classification system has more challenges and should be the one to be tested and used in this application.
The reviewer is forced to reject the manuscript, due to insufficient novelty and practical applicability.
Author Response
Please see the attachment.
Author Response File: Author Response.pdf
Reviewer 2 Report
1. Section 2 needs to be structured. It is better to introduce different clauses for speech separation and language identification algorithms. There are no quantitative estimates of the quality of the algorithms given in the Related Work. In Section 5, there is no comparison of the obtained results with the results of other authors.
2. The algorithm for mixing speech signals from datasets is not fully described.
3. It is necessary to provide a detailed description of the decision-making principles for language identification, especially for a mixed signal variant. Was there automatic segmentation in mixed signal analysis (e.g. 0.6 overlap)? If so, how was the correctness of the language identification in these segments assessed?
4. Why was it impossible to study speech separation and language identification algorithms on the same dataset?
5. Lines 358-359. Why are the parameter names repeated in parentheses? There is a typo - "recal1".
Author Response
Please see the attachment.
Author Response File: Author Response.pdf
Reviewer 3 Report
The paper presents a framework that utilizes single-channel speech separation network to improves the performance of downstream language identification task in multilingual overlapped speech scenarios. The results have shown the effectiveness of the proposed system. However, the paper is not very well written.
My further comments are as follows:
- In Section 5.1 and 5.3, there are experiments on using an overlap ratio of 1. How did you determine the correct labels? Because the language ID will be 50-50 between the original and the added one.
- Please proof-read the manuscript and carefully re-write it before re-submission. The current readability is very poor.
- Section I, Par. 1
... may not be able to separated and ...
--> ... may not be able to separate and ...
The neural network models brought ...
--> Neural network models brought ...
- Section II, Par. 1
Please describe what does "PIT" stand for.
- Section 3.1
It is mentioned that this paper uses Conv-TasNet from [33]. However, the original is [14]. What is the difference? Further, I also cannot find [33] from anywhere. The referred "Computer Application Research" does not seem to be existing.
- Section 3.2
In several sentences, "images" are used to describe the system architecture. Please use the proper term related to the proposed system functionality, i.e., image or speech.
- Section 4.1
Please provide reference to "AP20_OLR".
- Section 4.2.1
... where and represent the estimated mixed speech and ...
--> ... where $\hat{s}$ and $s$ represent the estimated mixed speech and ...
... respectively represents the signal power also represents the inner product.
--> I cannot understand what do you want to say. Please revise the sentence.
- Section 4.3
Please briefly describe what is cross-functional loss function.
- Section 5.1)
The description starting from "We replace the speech spectrogram ..." is very confusing, consisting of too many phrases and only within one sentence for about 8 lines of descriptions.
- Figure 6.
Caption is written on a separate page than the figure. It also has the same caption as Figure 7.
- Tables 3, 4, and 5, have the same captions. It is very confusing. Similarly for Tables 7, 8, and 9.
Author Response
Please see the attachment.
Author Response File: Author Response.pdf
Reviewer 4 Report
There are a lot of editorial flaws, e.g.:
1. There is an unusual citation way on lines 161-166. Instead of the authors' names, it appears the word „literature”, i.e. „literature [28,29,30] proposed an ….. In 2018, the literature [31] proposed an ….. In 2020, literature et al. [32]”
2. On line 268 should be “Figure 4” instead of „Figure 3”.
3. On line 290 „representation e” refers to formula (5), but there it is denoted by the letter c.
4. Incorrect is the definition of “softmax function” given by formula (4). What are W and T and what are their values?
5. On line 336 math symbols are missing in “where and represent”.
6. Why does the numbering format in Chapter 5 differ from that in other chapters, on line 378 “5.1)“, on line 407 “5.2)” and “5.1)” and on line 432 “5.3)”?
7. Why are the words „accuracy (Accuracy) and loss (loss)” duplicated on lines 419-420?
8. On line 465 is „A) The graphs” and should be „A) the graphs” similar like two lines above.
Author Response
Please see the attachment.
Author Response File: Author Response.pdf
Reviewer 5 Report
In this work, the authors attempt to combine the components of speaker separation and language identification. The related methods for each framework are based on the existing method and the aggregated work indeed boosts the empirical performance of language identification.
To further improve the paper, the authors should consider more ``selling points'' for this work. For example, the whole system consists of separately well-trained two components, but I was wondering if an end-to-end deep learning system, where a deep learning system for language identification is set up on top of another system for speaker separation. In particular, the 1d time-series signal should not be recovered by the decoding operation of speaker separation, and the step of spectrogram features extraction could be faithfully ignored. The authors may refer to the work [1] for the setup of experimental configuration and analyze the system based on the theoretical paper [2].
[1] Cai W, Cai D, Huang S, Li M. Utterance-level end-to-end language identification using attention-based CNN-BLSTM. In Proc. IEEE International Conference on Acoustics, Speech and Signal Processing (ICASSP), 2019, pp. 5991-5995.
[2] Qi, J., Du, J., Siniscalchi, S.M., Ma, X. and Lee, C.H., 2020. Analyzing upper bounds on mean absolute errors for deep neural network-based vector-to-vector regression. IEEE Transactions on Signal Processing, 68, pp.3411-3422.
Moreover, the authors may consider the comparison of different loss functions for the speaker separation system. For example, a simple mean absolute error (MAE) or distributional loss. The authors can refer to the papers [3] and [4].
[3] Qi, J., Du, J., Siniscalchi, S.M., Ma, X. and Lee, C.H., 2020. On mean absolute error for deep neural network-based vector-to-vector regression. IEEE Signal Processing Letters, 27, pp.1485-1489.
[4] Siniscalchi, S.M., 2021. Vector-to-vector regression via distributional loss for speech enhancement. IEEE Signal Processing Letters, 28, pp.254-258.
Additionally, the authors should take care of some minor mistakes in English usage, e.g., As Most of ... (line 12); and experimental setup in Section 3 ... (line 88), etc.
Author Response
Please see the attachment.
Author Response File: Author Response.pdf
Round 2
Reviewer 1 Report
The authors have answered my previous main concern, which was a misunderstanding from my side. Nonetheless, from a more careful reading of the paper and the described method, more questions have arisen that again make me reject the paper. More specifically,
> The effect of a source separator in the described setup is dubious. I mean the developed test set has the main speaker with a lot of higher energy than the background speakers, which means that the effect of the background is minimal and thus the job of the source separator is effortless. This can be testified by the reported SDR measurement of 15dB. No serious underdetermined source separation experiment I have seen so far can achieve an SDR of 15dB. This implies that the source separation is very easy and maybe a simple ICA algorithm and not a complex deep learning setup can also solve the problem. Thus the use of the proposed setup is dubious.
> Since the effect of the background speakers are so minimal, may the language ID network could have tackled the interference itself, without the use of the source separator. This should have been demonstrated in the experimental section in either case.
> Going to the Language ID section now, since the dataset offers 10 languages, why do you use only 5 in your experiments. This questions arises from the fact that your network can achieve extremely high levels of specificity (Table 9). This is may be due to something being wrong with your experiments, or the problem is extremely easy to solve by the network. Thus, you could have experimented with a more challenging problem and dataset.
Thus, the reviewer still recommends the rejection of the paper.
Author Response
Please see the attachment.
Author Response File: Author Response.docx
Reviewer 2 Report
All my comments have been corrected.
Author Response
Please see the attachment.
Author Response File: Author Response.docx
Reviewer 3 Report
Thank you for the response and revision. It looks fine.
Just one slight mistake on the last sentence of point 11, "vi-cn" should be "vi-vn".
Author Response
Please see the attachment.
Author Response File: Author Response.docx
Reviewer 4 Report
The work deserves publication. Congratulations!
Author Response
Please see the attachment.
Author Response File: Author Response.docx
Reviewer 5 Report
I think the revised manuscript is much better, but I also suggest that the publications I mentioned should be discussed and shown in the paper. Otherwise, the paper seems to deliberately miss some key points.
Author Response
Please see the attachment.
Author Response File: Author Response.docx