Artificial Intelligence, Machine Learning and Digital Innovation in Water Management
A special issue of Water (ISSN 2073-4441). This special issue belongs to the section "New Sensors, New Technologies and Machine Learning in Water Sciences".
Deadline for manuscript submissions: closed (20 September 2023) | Viewed by 18625
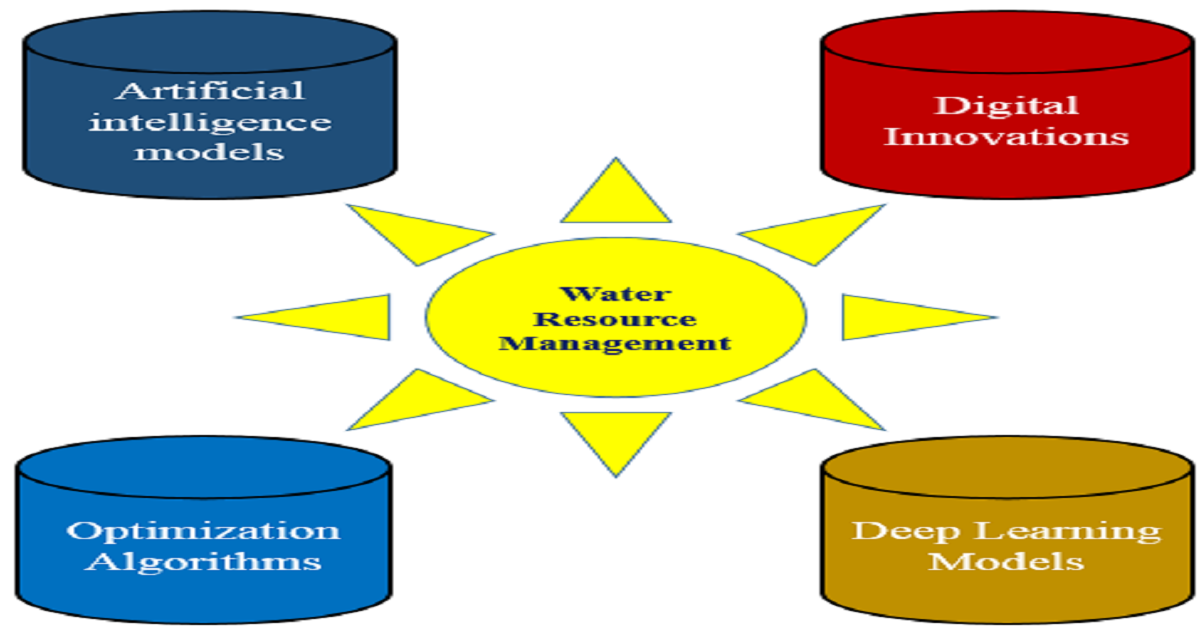
Special Issue Editor
Interests: water resource management and planning; soft computing models; climate changes; uncertainty modeling; artificial intelligence models
Special Issue Information
Dear Colleagues,
Today, water resource management is one of the most important topics for decision-makers, modelers, and policymakers. The management of water resources involves complex and nonlinear problems. Robust tools are required for solving and modeling optimization and simulation problems. Digital innovations, optimization algorithms, and artificial intelligence are robust models for managing and planning water resources. These models can be used to solve multidimensional problems with many constraints and objective functions. Machine learning and artificial intelligence models are useful tools for assessing the impacts of climate change on water resources. These tools can be integrated with remote sensing and geographic information systems (GIS) for planning and managing water resources.
The current Special Issue addresses the mentioned problems based on the following goals. However, the Special Issue is not limited to these topics.
- The application of artificial intelligence models and digital innovations for managing and monitoring water resources.
- The application of artificial intelligence models and digital innovations for predicting meteorological and agricultural parameters.
- Utilization of deep learning models for predicting natural hazards.
- Applying artificial intelligence models and digital innovations for predicting hydrological variables under climate change conditions.
- Spatial and temporal modeling of meteorological parameters using artificial intelligence models and digital innovations.
- Utilization of artificial intelligence models and digital innovations for solving complex problems in hydraulic engineering.
- The application of optimization algorithms for solving complex and nonlinear problems in water resource management.
- Utilization of optimization algorithms for optimal operation of dam reservoirs.
- The application of deep learning models and remote sensing for predicting hydrological variables .
- Quantifying uncertainty using machine learning models.
- Developing digital innovations for managing urban water systems.
- The application of ensemble models using the outputs of machine learning models for predicting hydrological variables.
- Stochastic environmental research and risk assessment using artificial intelligence models.
- Predicting climate patterns using artificial intelligence
Dr. Mohammad Ehteram
Guest Editor
Manuscript Submission Information
Manuscripts should be submitted online at www.mdpi.com by registering and logging in to this website. Once you are registered, click here to go to the submission form. Manuscripts can be submitted until the deadline. All submissions that pass pre-check are peer-reviewed. Accepted papers will be published continuously in the journal (as soon as accepted) and will be listed together on the special issue website. Research articles, review articles as well as short communications are invited. For planned papers, a title and short abstract (about 100 words) can be sent to the Editorial Office for announcement on this website.
Submitted manuscripts should not have been published previously, nor be under consideration for publication elsewhere (except conference proceedings papers). All manuscripts are thoroughly refereed through a single-blind peer-review process. A guide for authors and other relevant information for submission of manuscripts is available on the Instructions for Authors page. Water is an international peer-reviewed open access semimonthly journal published by MDPI.
Please visit the Instructions for Authors page before submitting a manuscript. The Article Processing Charge (APC) for publication in this open access journal is 2600 CHF (Swiss Francs). Submitted papers should be well formatted and use good English. Authors may use MDPI's English editing service prior to publication or during author revisions.
Keywords
- artificial intelligence models
- digital innovations
- hydrological simulations
- meteorological predictions
- optimization algorithms
- deep learning models
- natural hazard
- hydraulic problems
- irrigation and agriculture management