Artificial Intelligence (AI) in Radiation Oncology
A special issue of Journal of Personalized Medicine (ISSN 2075-4426). This special issue belongs to the section "Methodology, Drug and Device Discovery".
Deadline for manuscript submissions: closed (5 May 2023) | Viewed by 6518
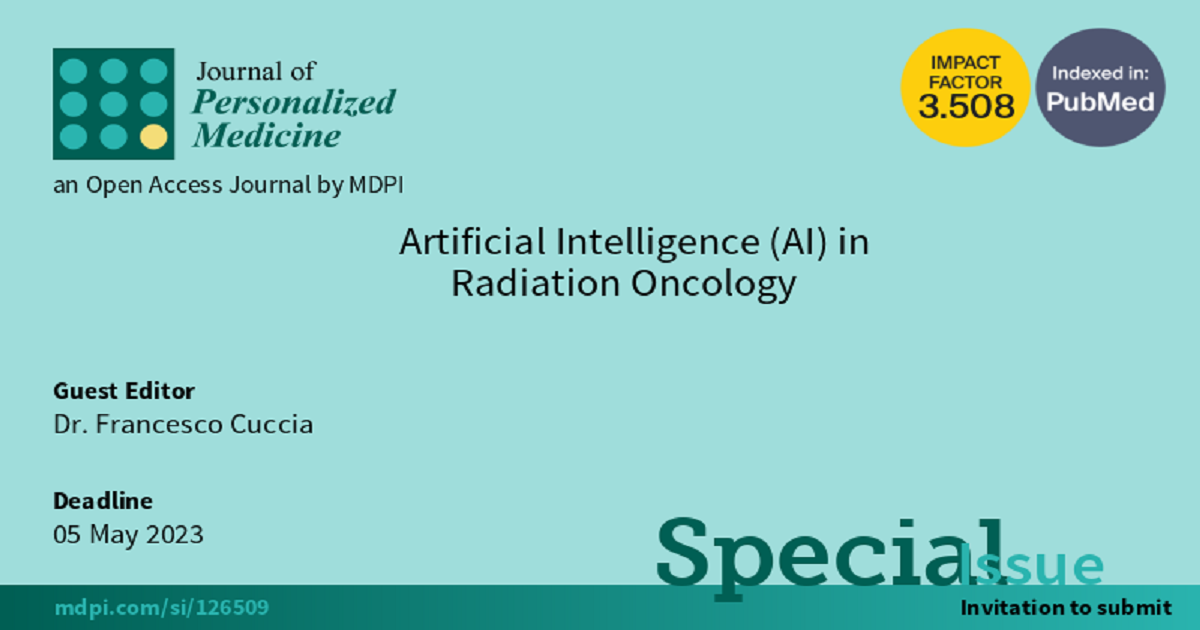
Special Issue Editor
Interests: radiation oncology; radiotherapy physics; stereotactic radiosurgery; intensity-modulated radiotherapy; adaptive radiotherapy; lung cancer; prostate cancer
Special Issues, Collections and Topics in MDPI journals
Special Issue Information
Dear Colleagues,
Technological advancements are leading the way in the field of radiation oncology, with the recent introduction of new hybrid MR-guided linear accelerators and the global widespread of daily adaptive radiotherapy. Alongside a deeper knowledge of cancer biological and genomic signatures, there is a growing amount of data available from imaging exams with radionics that should allow clinicians to gain new information in terms of outcome prediction. In this scenario, the role of artificial intelligence is gaining attractiveness in the scientific community as a helpful tool for both refining accuracy in treatment delivery and improving knowledge about predictive factors for clinical outcomes.
The aim of this Special Issue is to cover novel innovative findings and concepts within the field of artificial intelligence applied to all aspects of radiation oncology.
Dr. Francesco Cuccia
Guest Editor
Manuscript Submission Information
Manuscripts should be submitted online at www.mdpi.com by registering and logging in to this website. Once you are registered, click here to go to the submission form. Manuscripts can be submitted until the deadline. All submissions that pass pre-check are peer-reviewed. Accepted papers will be published continuously in the journal (as soon as accepted) and will be listed together on the special issue website. Research articles, review articles as well as short communications are invited. For planned papers, a title and short abstract (about 100 words) can be sent to the Editorial Office for announcement on this website.
Submitted manuscripts should not have been published previously, nor be under consideration for publication elsewhere (except conference proceedings papers). All manuscripts are thoroughly refereed through a single-blind peer-review process. A guide for authors and other relevant information for submission of manuscripts is available on the Instructions for Authors page. Journal of Personalized Medicine is an international peer-reviewed open access monthly journal published by MDPI.
Please visit the Instructions for Authors page before submitting a manuscript. The Article Processing Charge (APC) for publication in this open access journal is 2600 CHF (Swiss Francs). Submitted papers should be well formatted and use good English. Authors may use MDPI's English editing service prior to publication or during author revisions.
Keywords
- artificial intelligence
- radiation oncology
- adaptive radiotherapy